With modelling advice from Dr Nishaal Gooroochurn, Oxford Economics
Chapter 1
The government has set out plans for a staged re-opening of different parts of the economy as the country emerges from lockdown. But if easing goes too far, the spread of the virus could begin to accelerate again, risking thousands more lives and a potentially more severe second economic shock. To make matters worse, we have no clear sense of the likely impact of different easing steps on the reproduction number, R. And since the virus spreads rapidly, and unseen at first, we will only learn of any missteps long after they have been made.
This paper proposes a Covid-19 early-warning model to help policymakers navigate this perilous terrain. By allowing them to forecast and monitor the effect of easing measures on the spread of the virus in real time, such a tool could help us manage the virus and minimise the cost at a national and local level.
We use an econometric model to identify the relationship between physical mobility and the subsequent spread of the virus, using data provided by Google at upper-tier local authority level for England. From mid- to late March, the data shows that mobility across retail, workplaces and public transport in the UK dropped to around 40 per cent of its normal level as the government imposed a strict lockdown. As a result, the rate of acceleration of the virus began to fall, and went negative in early April, consistent with epidemiological estimates that R has been below the critical level of 1.
Our model suggests that a one percentage point increase in mobility leads to a 2.2 percentage point change in the acceleration of the virus. Applying this relationship to the recently observed numbers of new cases in England suggests that mobility could recover to around 51 per cent of its normal level without causing R to breach the threshold of 1. This would represent a significant increase in economic activity, potentially saving many businesses and jobs.
At a local level, we find that some areas of the country have substantial headroom to increase mobility safely, while a minority need to restrict mobility further to slow the spread of the virus. London, Merseyside, the West Midlands and Bristol could all increase mobility by around ten percentage points, but in Slough and York it appears already to be too high to get R below 1 locally.
Monitoring these mobility levels in real time could allow different parts of the country to take steps to increase or decrease mobility in their area, optimising wellbeing within a total “mobility budget”. Using the early-warning system in this way would:
Maximise economic activity without the virus running out of control, thus saving many jobs and businesses.
Allow quicker intervention to stop the spread of the virus, maximising the health benefits and minimising the economic damage.
Facilitate locally targeted measures to keep the virus under control without the need to resort to heavy-handed national restrictions that would be unnecessarily costly in areas unaffected by an outbreak.
Allow real-time experimentation, so that the whole country can learn from the experience of different areas as new containment measures are rolled out.
As containment infrastructure is developed, or as immunity increases in the population, the model could be re-estimated to find the new safe level of activity. Such a tool could help us to reopen the economy as quickly as it is safe to do so.
Chapter 2
Lockdown has saved thousands of lives by bringing the spread of Covid-19 under control in the UK. However, it is increasingly clear that harsh suppression measures are unsustainable, as the economic, social and indirect health costs mount. They need to be eased soon if we are to prevent severe long-term economic and health damage.
In its roadmap for lifting the lockdown measures, the government has set out three steps of easing, starting with opening workplaces for those who can’t work remotely, moving on to opening shops and schools, and finally reopening restaurants and cafes at some point in July. But if easing goes too far, it could restart the epidemic, causing thousands more deaths and a second economic shock from another lockdown, the consequences of which could be worse than the first.
At the same time our ability to navigate the narrow path between these two catastrophic outcomes is heavily circumscribed. We have no clear sense of what will happen to the rate of spread of the virus as different easing steps are taken alone or in combination, nor of the behavioural response of a lockdown-weary nation perhaps believing that the danger is past. And since the virus spreads rapidly before evidence emerges, in the form of positive test results, we will only learn of missteps many days after they have been made.
In short, we are embarking on the journey with a sketched map and a faulty steering wheel, and only able to navigate using the rear-view mirror.
With so much resting on the decisions ahead, policymakers need tools that can help them quantify the risks of easing measures in advance and give them real-time feedback on their consequences so that errors can be corrected quickly.
To help achieve that goal, this paper sets out a Covid-19 early-warning system that could alert decision-makers to the likely rate of spread of the virus just hours after easing measures come into effect and in granular geographic detail. Based on population mobility data, we set out to identify the relationship between movement and the subsequent spread of the virus at local authority level across England. The relationship uncovered is then applied to the current rate of spread of the virus in each area to estimate the scope different places have to increase the amount of mobility safely, and therefore economic activity.
Chapter 3
For much of March the UK public was largely unconcerned about the approaching epidemic, or at least not sufficiently concerned to reduce their trips to restaurants, shops and workplaces – the stuff of economic activity – which proceeded at normal levels. And largely as a result of this normal, high level of mobility, the virus spread rapidly, and largely unseen, through the population, with R estimated by Imperial College to have been as high as 3.9 (Figure 1).[_]
Early March: High mobility = high GDP = high R
The lockdown was explicitly introduced on 24 March to stop people moving and coming into contact with one another, in order to break the chain of transmission. The weight of policy was effectively shifted from maximising economic activity to minimising the spread of the virus. What inevitably flowed from these heavy restrictions on mobility was that many people were unable to work, and everyone was prevented from being a customer in bars, hotels, restaurants and most shops. As a result, economic activity fell by around one-third as a direct result of the public health need to stop moving around, and the R number under lockdown appears to have fallen to around 0.7 (Figure 2).[_]
April: Low mobility = low GDP = low R
But this situation is unsustainable as the indirect health costs, economic costs and social costs of lockdown mount, hence the government’s exit roadmap. Even somewhat higher mobility could raise economic activity, saving thousands of jobs, businesses and lives for other reasons. Hence the optimal exit from lockdown – one that maximises societal wellbeing – involves maximising mobility subject to keeping the R number below 1. The prize for achieving such a well-managed, balanced exit is large.
Smart exit: Optimal mobility = higher GDP = R just below 1
Walking this optimal path is extremely difficult for two main reasons. First, we don’t know exactly what impact any given easing measure will have on the spread of the virus. Would opening small shops keep R below 1? What impact would opening schools have? While some broad estimates are available for some of these steps, we have little sense of the relative consequences of the many different components of a phased exit, and waiting to learn from the experience of other countries will be too slow.
The second problem is that the virus spreads rapidly for days before it shows up in rising numbers of positive tests, and much longer before the number of deaths starts to rise. While symptoms tend to appear after five or six days, it can take up to two weeks, there are inevitable delays in testing and reporting, and the proportion of asymptomatic cases appears to be high.[_], [_] These attributes mean that by the time it becomes clear that the virus has slipped out of control, it is likely that a region may have a serious outbreak on its hands, and a harsh lockdown may then be the only way to contain it. While there are emerging attempts to measure R at a local level, these models rely on data on deaths that involve a considerable time lag, limiting their use in monitoring the immediate effects of easing.[_]
Chapter 4
Since the virus spreads faster the more mobile the population is, this raises the possibility that mobility levels could be used to create a real-time, visible proxy for its spread. Understanding the relationship between mobility and the rate of spread would also make it possible to estimate the optimal level of mobility that maximises economic activity while keeping the epidemic under control: the “mobility budget” if you like (Figure 4).
Figure 4 – The trade-off between mobility and R
What’s more, a virus-forecasting tool would allow the authorities to take targeted local action to dampen population mobility and pre-emptively reduce the spread of the disease, rather than later having to slam on the economic brakes at higher cost.
This could be valuable at local-authority level. If, say, Hampshire has a declining number of cases under lockdown, when mobility is around 40 per cent of normal levels, then it may be possible to identify a higher level of mobility that would still be consistent with controlling the virus, and keeping R below 1.
There would be other benefits too. Such a tool would make it possible to see the likely impact of, say, opening small shops or schools on the mobility level in one area in order to inform the strategy in other areas. It would also allow us to compare the relative merits of opening different kinds of institutions: Does opening some workplaces use up a disproportionate amount of the mobility budget compared to others?
Meanwhile if areas in the vanguard of reopening, those exhibiting higher mobility, were able to demonstrate that they can control the spread of the virus through effective containment measures, the forecasting model could be re-estimated to divine the new “safe” level of nationwide mobility. Rather than embarking upon one massively risky national experiment, this would allow safe learning and experimentation that could save thousands of lives and livelihoods.
This approach could be used at national level, to decide whether to tighten national restrictions, or it could be used by local authorities: If one local authority’s early-warning light is flashing red because mobility is above the critical level, it could decide to take locally targeted measures to dial it down, or message inhabitants asking them to avoid unnecessary shopping, without having to stop everyone else from working.
There are therefore numerous potential benefits of successfully quantifying the link between mobility and the rate of spread of the virus. In the rest of this paper we outline our attempt to achieve that goal using an econometric model and data from Google.
Chapter 5
Google produces data on population mobility each day based on mobile-phone usage. For the UK these data are also disaggregated by local authority, and by type of organisation, including retail outlets, grocery shops, workplaces and public transport. Google provides estimates of activity in these establishments relative to a benchmark of “normal” levels of movements, defined as the median reading on the days between 3 January and 6 February 2020.[_]
We used the data to create a combined average of the retail, grocery, workplace and public-transport mobility levels. We leave aside the data series on activity in parks and residential spaces, which has obviously increased markedly in recent weeks, on the grounds that these types of mobility are not associated with the community transmission that lockdown was intended to address. Plotting this measure reveals that mobility levels in the UK remained close to normal levels right up until 17 March, when the government’s recommendation for people who could to work from home came into effect. Activity dropped further in the wake of the announcement of full lockdown, on 23 March, finding a floor with mobility at just under 40 per cent of normal levels (Figure 5).
Figure 5 – Mobility in the UK relative to normal, March to May 2020
When it comes to the spread of the virus, Public Health England produces local authority-level data on the number of cases reported each day from the Pillar 1 NHS testing regime. We can use the time series of cumulative new cases in each area to develop a measure of the rate of acceleration of the spread of the virus. Both because the daily reporting of test results is lumpy, and because the typical incubation period of the disease is around five days, we use the data to develop a five-day acceleration rate: the number of cases reported in the last five days as a proportion of those in the five days before that.
This measure means that, were the number of new cases in one five-day period to be twice the level in the the previous period, the acceleration of the virus would be 100 per cent. Meanwhile if the number of new cases remains constant from one period to the next, acceleration would be zero. Zero acceleration is equivalent to R being equal to 1.
We can expect changes in mobility to have an immediate impact on the rate of spread of the virus, since people come into contact with fewer other people when they move around less. But that impact takes time to show up in the daily reported case numbers, and hence in our five-day acceleration measure. Given the typical incubation of the virus and the inevitable lag in getting a confirmed test result after the onset of symptoms, we use a lag between changes in mobility and changes in case numbers of five to ten days. This lag appears to fit the data well (see appendix).
There are clear limitations with using confirmed case numbers. First, the availability of tests and changes in testing policy can influence the figures. It is also the case that the number of confirmed cases from Pillar 1 testing is only a proportion of total infections occurring in the population. All of this means that the number of confirmed cases is only an imperfect measure of how the virus is spreading but, we believe, a reasonable one (see below for further discussion).
Comparing the data series in different areas of the country, we can see a clear relationship between falling mobility due to the lockdown and the subsequent rapid decline in acceleration of the virus, to the point where the spread is decelerating (Figure 6). This is consistent with the epidemiological estimates that R is currently less than 1.
Figure 6 – Relationship between mobility and the rate of spread of Covid-19
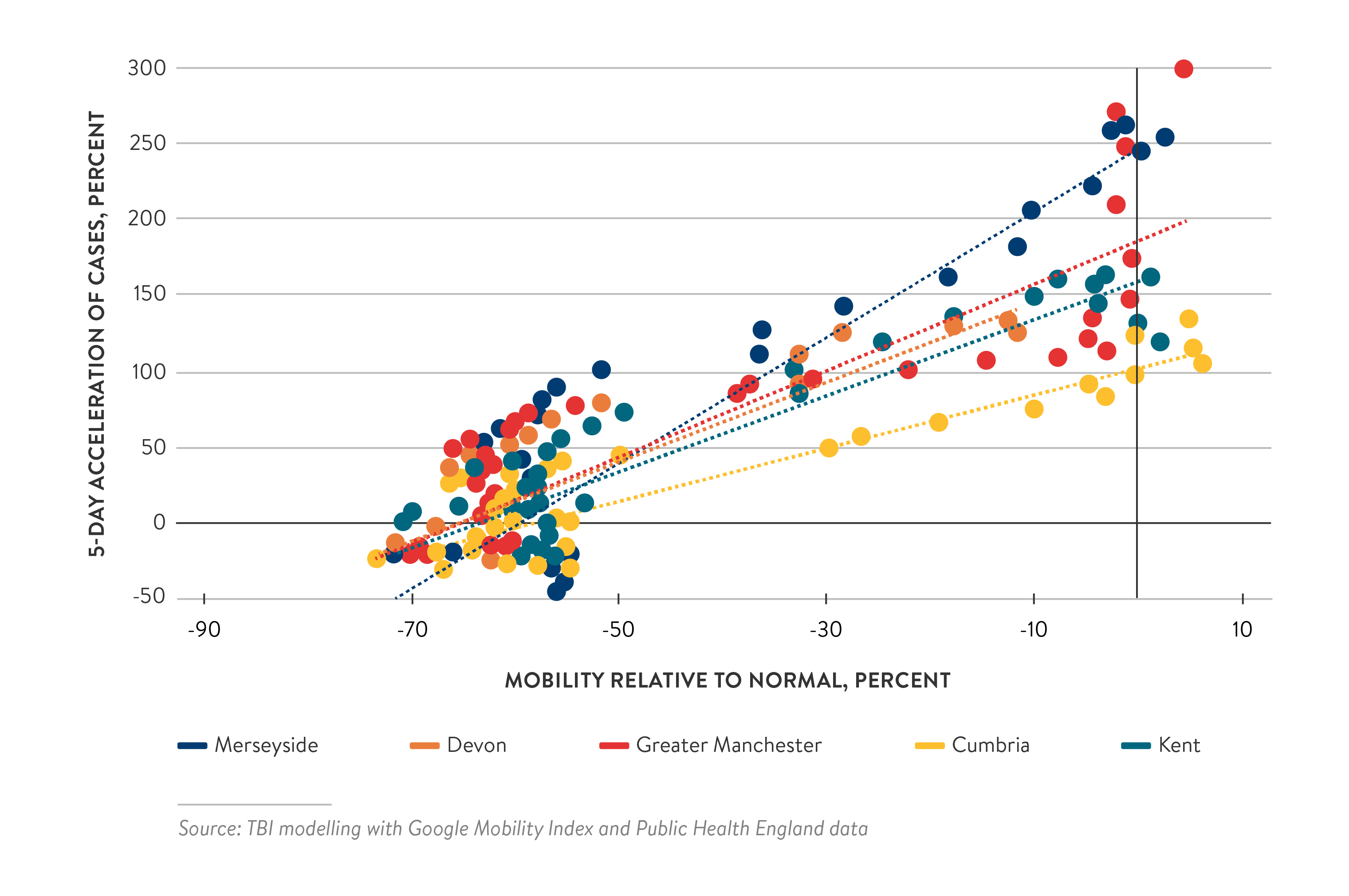
Having developed these two series at upper-tier local-authority level for England, we built an econometric model to relate the mobility data to the growth rate in the number of cases and establish how much the spread accelerates for a given increase in mobility. Details of the modelling approach and robustness checks are provided in the appendix.
Chapter 6
The main variant of the model suggests that a one percentage point change in the level of mobility is associated with a 2.22 percentage point change in the acceleration of the spread of the virus (95 per cent confidence interval 2.00 to 2.45). During the first seven days of May, mobility in the UK ran at about 44 per cent of its usual level, and the spread of the virus was slowing, at a rate of around -21 per cent per five-day period. Applying the measured relationship for England to the national average suggests that mobility could increase by around seven percentage points, to reach 51 per cent of normal, without the spread of the virus accelerating – without R breaching the critical level of 1 (Figure 7). It is notable that in recent days mobility has drifted upwards towards the threshold.
Figure 7 – Implied mobility ceiling based, UK average
This implies that the UK has some headroom to ease restrictions, but that anything approaching normal levels of activity would require significant offsetting containment measures sufficient to alter the relationship observed here.
Chapter 7
These results can be applied to the observed rate of spread of the virus at local level, to get a sense of how much each area can afford to increase the level of mobility without the R number going above 1 (Figure 8). Doing this for the different areas of England shows that there are a number of areas that could safely accommodate mobility levels around ten percentage points higher than they are today. These include Greater London, Merseyside, the West Midlands and Bristol, for example.
At the same time, there are 14 areas where the spread of the virus appears still to be accelerating, and where mobility could be reduced to try to bring those outbreaks under control. Some of these areas are York, Slough and the Isle of Wight. As we might expect, the ten areas with the most rapidly falling spread have mobility levels currently around five percentage points lower than the ten areas with the most rapidly accelerating spread.
Figure 8 – How much can different areas safely increase their mobility?
Monitoring these mobility levels in real time could allow different parts of the country to take steps to increase or decrease mobility in their area in order to keep the rate of spread of the virus under control. This would have a number of advantages:
Hitting the “mobility budget” in each area would maximise economic activity without the virus running out of control, thus saving many jobs and businesses.
Rather than waiting to see if cases rise, with the inevitable delays that entails, the early-warning system could allow quicker intervention to stop the spread of the virus, maximising the health benefits and minimising the economic damage.
Activity could be fine-tuned in different local areas to keep the virus under control without the need to resort to heavy-handed national restrictions that would be economically costly in areas unaffected by an outbreak.
Finally, the model would allow real-time experimentation, so that the whole country can learn from the experience of different areas and further minimise the risks associated with easing the lockdown.
Chapter 8
As well as gradually easing the lockdown restrictions, the government’s intention is to build up its containment infrastructure. Containment includes any measures that inhibit the spread of the virus in ways other than reducing mobility, such as tracing and testing infrastructure, and recommendations that people wear face coverings.[_]
Effective containment will take some time to develop, but as it starts to take effect it will allow higher – and eventually hopefully close to normal – levels of mobility without the virus spreading. This means that the relationships estimated above should change over time such that one percentage point more mobility increases the acceleration rate by less than 2.2 percentage points as things like testing and tracing programmes expand (Figure 9).
Figure 9 – Effective containment measures will reduce the sensitivity of R to mobility
The results of the current model are therefore likely to imply optimal mobility levels that err on the side of caution if we think that containment is already better than it was in late March and April. As containment improves it would be important regularly to re-estimate the model and update these local “mobility budgets”.
Chapter 9
The results above suggest that a mobility-based Covid-19 early-warning system could be useful in optimising our exit from lockdown, saving lives and livelihoods. But there are limitations with the approach outlined here, some of which could be improved upon.
Use of confirmed case numbers. As discussed above, the model is based on case numbers confirmed by positive Pillar 1 tests, which could be an unreliable proxy for the wider spread of the virus. This should not present a major concern to the extent that Pillar 1 tests have been administered according to a broadly consistent policy over the past two months. As a check, we can compare the rate of decline in the number of daily deaths with that of the Pillar 1 confirmed cases. The two series have declined at a roughly similar rate with both metrics down by a little over half since their peaks.
Lags and transmission. The fact that the virus spreads within confined spaces means that there can be long lags between changes in mobility and new infections, as the virus spreads through households or institutions like care homes. This means that estimates of the relationship are uncertain, and it is unlikely that all cases triggered by a given day’s mobility will be captured within the lag we have used in this model. This should lead to a conservative estimate of the relationship, which has the advantage of erring on the side of caution in easing lockdown.
Immunity. There remains uncertainty about whether and for how long infected people are immune to contracting Covid-19, but the working assumption from many experts seems to be that some immunity is likely to be conferred. If so, then as the virus spreads through the population, the R associated with normal levels of mobility will tend to fall even with no containment measures in place because of the insulation provided by people who are immune. This will tend to lower our estimate of 2.2, hence it will be important to re-estimate the model at regular intervals to identify any changes in the relationship.[_]
Random variation. Research into how Covid-19 spreads is in its early stages, but from migrant worker dormitories in Singapore to care homes in the UK, it appears that infections within institutions can trigger rapid spread of the virus even if wider community mobility is low. Consequently, mobility is far from a perfect predictor of the spread of the virus within a local area, but it should influence the risk of suffering such an outbreak.
Chapter 10
This paper has proposed a model linking mobility to the rate of transmission of Covid-19, and developed a prototype, as a forecasting tool to maximise economic and other social activity while keeping the virus under control. By providing a real-time indicator of the rate of spread of the virus, such a model could offer early warning of the acceleration of the virus, local targeting of measures to slow its spread, and the potential quickly to learn what works from parts of the country that open up first so that we reopen the economy as quickly as it is safe to do so.
There are inevitable limitations to the data on which such a model is built, which limits its accuracy. It may also be the case that others can propose improvements to the modelling approach used here. Nevertheless, this paper has set out an approach that may merit further development into a policy tool given what is at stake as we learn to live with Covid-19 in the months ahead.
Chapter 11
The aim of this modelling exercise is to estimate the relationship between the levels of physical mobility across society and the rate of spread of the virus. Establishing a relationship between the two quantitatively can provide a valuable yardstick for policymakers to assess, real time, the impact of easing lockdown restrictions and pre-empt further outbreaks.
Data
To develop the outcome variable, the rate of spread of the virus, we use data on coronavirus cases in England by upper-tier local authorities provided daily by the UK government.[_] The cases correspond to positive tests carried out under the government’s Pillar 1 testing regime that includes “swab testing in Public Health England (PHE) laboratories and NHS hospitals for those with medical need and for critical workers and their families/household members”[_] and excludes Pillar 2 tests carried out by commercial partners for key workers and their households. Pillar 2 tests have expanded substantially in scale since the early days of the outbreak, making them a poor proxy for the rate of spread of the virus, and to date the results are not geographically disaggregated.
To understand how quickly the virus is spreading we use cumulative cases reported to calculate acceleration as:
Where subscript LA,t denotes an observation for a given local authority at date t and t – 5 for a date five days prior to that. Δ5,t refers to the five-day growth in cumulative cases at date t (i.e. the number of new cases recorded in those five days). Our acceleration measure captures the rate of change of that five-day growth Δ5,t. To illustrate, an acceleration of zero on 15 April would mean the number of new cases recorded between 10 and 15 April was the same as that between 5 to 10 April. On the other hand, an acceleration rate of -100 per cent would mean there were no new cases in the last five days, so the cumulative number of cases on 15 April remained the same as on 10 April. Intuitively, positive levels of acceleration relate to a reproduction number (Rt) greater than 1 whereas negative acceleration indicates Rt is less than 1.
There are several reasons why growth in cases, on which we base our acceleration calculation, might not be reflective of the true underlying trajectory of the virus. For example, there seem to be consistently fewer cases reported over the weekends followed by a “catch-up” spike on Mondays. Variability in testing over time, and possibly across some local authorities, will also introduce fluctuations in the reported number of cases that may obscure the virus’s real trajectory. Finally, the inevitable lag in registering and reporting causes an artificial drop in case numbers in the last few days of the time series. To address these problems, we drop the last three days in the sample and smooth the underlying five-day growth in cases by taking a four-day moving average.
However, acceleration in cases might still be unusually volatile for reasons that smoothing of the case data might not address. For small numbers of cases in early phases of the outbreak, a reported growth from one to 11 cases followed by a growth of three would result in a series of accelerations of 1100 per cent to 72 per cent respectively. These sunspot fluctuations in cases at low case numbers are a problem for observations at the beginning of the outbreak and occur most in local authorities still at early stages of their outbreaks and in rural, sparsely populated areas where testing and reporting may be less consistent. To address the challenge of noisy data when case numbers are low, we take two steps: we only use data for areas after they reach a cumulative total of 20 cases, and we drop the 24 least-affected local authorities by using data only for those areas that reached a 350 total number of cases by 30 April. The result is a data set covering 64 upper-tier local authorities and metropolitan areas.
To capture the impact of the lockdown we use the mobility index data provided by the Google COVID-19 Community Mobility Reports.[_] The mobility data provided capture how visits and length of stay at different places change, relative to a baseline of normal activity, at upper-tier local authority and metropolitan-area level across the UK. The data capture mobility across six different types of places:
Grocery & pharmacy; e.g. supermarkets, food warehouses, farmers markets, speciality food shops and pharmacies
Parks; e.g. local parks, national parks, public beaches, marinas, dog parks, plazas and public gardens
Transit stations; e.g. public-transport hubs such as tube, bus and train stations.
Retail & recreation; e.g. restaurants, cafes, shopping centres, theme parks, museums, libraries and cinemas
Residential
Workplaces
For our purposes we exclude mobility changes in parks and places of residence, which we expect to increase during the lockdown, and are not expected to play a (significant) role in the community transmission that lockdown was intended to reduce. We therefore calculate a daily mobility score for each upper-tier local authority and metropolitan area as an average of the remaining four elements:
Changes for each day are compared to a baseline value for that day of the week. The baseline is the median value for the corresponding day of the week, during the five-week period from 3 January to 6 February 2020. For example, a mobility figure of -60 per cent in London on Thursday 14 April 2020 can be interpreted as there being on average 60 per cent fewer people that day across London’s train stations, shops, grocery markets, offices or cinemas compared to a typical Thursday in London between 3 January and 6 February. Since the data are collected from mobile phones, the series are based on the activity of users who have opted in to Location History on their Google account, so the data represents a sample of smartphone users rather than all. However, so long as smartphone users who have opted in to Location History are no more or less likely to stay at home than anyone else, these data will give an accurate representation of the change in mobility in each location.
We compile a panel dataset of 79 Upper Tier Local Authorities and 7 Metropolitan areas spanning from 15 February to 6 May.
Econometric Modelling
We estimate a model of acceleration of Covid-19 cases as a function of past mobility. A study by researchers at Imperial College London using Chinese data suggests there is a mean interval of 6.48 days between catching the virus and showing symptoms with a standard deviation of 3.83 days, and an additional four days on average between showing symptoms and getting tested.[_] While the UK testing interval may vary, we would expect changes in mobility to affect the number of new cases with a lag of at least seven days and at most two weeks, by which time a given change in mobility is expected to have had full effect.
We assume, for a given level of mobility, there are differences in acceleration between local authorities that are fixed over time (uLA). These could reflect differences in density or industrial structure meaning different proportions of the workforce employed in high proximity industries or even differences in demographic characteristics that lead to varying propensities to adhere to social-distancing restrictions or exercise extra caution.
Keeping mobility constant, case acceleration is also likely to fluctuate between local authorities over time (ϵLA,t). We assume these stochastic fluctuations are mean zero, such that the model is not systematically over- or under-predicting acceleration, and are uncorrelated with mobility such that the estimated coefficient β captures its true effect on the spread of the virus. We allow, however, for the variance of those random disturbances to vary across local authorities and over time, by using heteroskedasticity robust standard errors in estimation.
Estimation Results
We estimate a fixed effects panel model using data for 81 upper-tier local authorities and seven metropolitan areas. As described above, we only use local authorities where the case data is less prone to volatility by dropping areas with fewer than 350 cumulative cases by the end of April. We further restrict the sample for each of these local authorities so as to start only once it has reached a total number of 20 cases or more. We do not include the last five days in the dataset. Due to a lag in reporting, the last five observations are often revised upwards as new data become available, as indicated on the PHE website, making these figures unreliable.
The estimated results of our central model are presented below:
The estimated coefficient is 2.2 and significant at the 1 per cent significance level. This means a one percentage point increase in mobility is expected to increase five-day acceleration by 2.2 percentage points after ten days. For mobility at normal levels, (in the Google index equivalent to a mobility of zero), we estimate a five-day acceleration of 132 per cent.
How does this compare to epidemiological estimates of R0? It is hard to be precise, but a broad sense check is possible. For an infectious period of five to seven days this acceleration is roughly consistent with R0=[2.3,3.2][_] , comparable with the range of epidemiological estimates of R0 for the UK.[_] What do the results imply for a situation where mobility was reduced to zero? Reducing mobility from its third week of April average of -60, by 40 points (i.e. to -100), would, according to our model, result in a drop in acceleration of 88 percentage points. Given that end of April national acceleration was roughly -22 per cent, the model correctly predicts that completely ending mobility would, after a lag, bring about a complete halt in number of new cases, as we would expect. This is equivalent to Rt=0.
The validity of our results relies on the population immune to the virus and the containment technology available being broadly stable across the sample. The model also assumes the relationship between acceleration and mobility is linear and the impact of mobility across regions, homogeneous. On the assumption of homogeneity, as shown in the sensitivity tests presented in the section below, the inclusion or exclusion of different regions from the estimation does not drastically alter the coefficient.
Comparison With Other Papers
On 4 May, researchers at Imperial College London published a paper which also uses mobility to forecast the reproduction number under different lockdown-easing scenarios for regions in Italy.[_] The authors use a semi-mechanistic Bayesian hierarchical model fitted to regional death-toll data, in which the reproduction number is parameterised using Google mobility data, so that the underlying rate of infections is fitted to the observed death toll as a latent function of that mobility. The same method is also used in another recent study by researchers at Stockholm University and the Science for Life Laboratory to estimate R0 and Rt for 11 European countries.[_]
Similar to the approach used in this paper, the validity of the Imperial and Stockholm University results relies on only a limited proportion of the population having acquired immunity, and the models assume that containment technology is constant. Also, both the Imperial model and Stockholm University's cross-country variant are jointly fitted in all regions. Both find the impact of residential mobility, which we exclude from our calculations, to be small or insignificant.
While direct comparison is difficult, the results from these exercises appear to be broadly similar to the findings presented here. The Imperial paper's scenario forecasts suggest that “even a 20% return to pre-lockdown mobility could lead to a resurgence in the number of deaths”. Consequently, their paper recommends that mobility be closely monitored when restrictions are eased and suggests that any easing can only be considered if it can be compensated with increased containment measures. The parameter estimates in this paper also appear comparable to the Stockholm paper's estimates of R0 and Rt for the UK under reasonable assumptions.
Robustness Tests
As noted earlier, we estimate the model using fixed effects to account for differences in acceleration between local authorities that are fixed over time and correlated with mobility. We test that assumption against that of random effects using a heteroskedasticity robust Hausman test, the results for which are presented below:
For robustness we check how the results vary if we ease the cumulative case cut-off points, the smoothing window, the acceleration window or use different lags. To reiterate, the selected model uses a five-day acceleration window smoothed over four lags and four leads (which we denote as 44)[_], has a ten-day lag, does not include places with fewer than 350 cumulative cases by 30 April, and excludes observations for which the total number of cases at any given date was lower than 20. For ease we denote the results corresponding to our central model in each table with asterisks.
Ideally, we would aim to capture the marginal impact of mobility for each day in the two-week impact window. Due to multicollinearity in neighbouring lags, identification of marginal effects is not possible. The table below shows how results vary by lag length:
Reducing the smoothing window amplifies the “sunspot” fluctuation at the beginning of the sample. For that reason we raise the cut-off to 80 cases for the results in the table below.
We also vary the window of acceleration, which varies the coefficients as expected.
Given that both the acceleration and mobility variables are trending and show signs of non stationarity, it is possible for the relationship found to be spurious. To reject spuriosity, we test this hypothesis using a panel cointegration test, examining whether the error term is stationary. We used the Kao (1999),[_] Pedroni (1999, 2004),[_] and Westerlund (2005)[_] tests with different lags and trend options, and found strong evidence of cointegration, implying the relationship we are estimating between acceleration and mobility is not spurious. The estimated coefficients are thus efficient and consistent. The p-values of these tests are summarised in the tables below: