Our Future of Britain initiative sets out a policy agenda for governing in the age of AI. This series focuses on how to deliver radical-yet-practical solutions for this new era of invention and innovation – concrete plans to reimagine the state for the 21st century, with technology as the driving force.
Governing in the Age of AI: A New Model to Transform the State is a joint report by the Tony Blair Institute for Global Change and Faculty.
Contributors: Benedict Macon-Cooney, Jeegar Kakkad, Roger Williams, Rachel Irwin, Ursule Kajokaite, Laura Britton, John Gibson, Nijma Khan, Paul Maltby, Sona Hathi-Rehman, Filip Wolski
Chapter 1
There is little doubt that AI will change the course of human progress, much like previous general-purpose technologies that dramatically reshaped the world around them. But unlike past waves of change, much of the foundational infrastructure for AI is already in place: the internet and data, cloud and storage, chips and compute. The scope and scale of change will be vast. And it will come quickly.
The private sector is already making historic investments in its future. With chips and data centres, leading tech companies are building infrastructure that surpasses 20th-century mega-projects such as railroads, dams and even space programmes. But across the corporate world leaders all face a choice: invest in AI capabilities or risk perishing.
For governments, the choice often feels less stark. Political leadership may change, but the state still exists. Like all well-established organisations, the state has a bias towards caution. But this is an illusion – a failure to modernise, reform and deliver is a perilous course for a nation and those who govern it. And this is particularly true in the case of AI, which if gripped properly, should make today the most exciting and creative time to govern.
In writing this paper, we are coming at this issue from both perspectives. One of us is a politician and runs an Institute advising government leaders, while the other is a leader of a technology company. We both understand the magnitude and the necessity of the choice. We both also see the potential prize for the UK, which should have its own ambitions to position itself at the forefront on AI and provide leadership on governing in this new era.
And when both of us survey the operations of governments from our different perspectives, we see the same opportunity: almost everywhere AI can help us reimagine the state. Many of the countless daily tasks in government are repeatable processes carried out on a mass scale.
Almost all of these can be made better, faster and cheaper. As this paper lays out, the scale of this opportunity is huge: with the technologies and the digital infrastructure we have today, we estimate that up to £40 billion can be saved each year with the technology as it exists now. But, of course, over time, this technology will accelerate dramatically in its capability, and so will the savings.
This is much more than a debate around the margins of tax and spending; it has the potential to transform the costs, functions and accountability of government.
At a time when government is unwieldy, expensive and slow, AI can save our public services, making them more personalised and human-centric.
Safe, explainable AI systems can make government fairer and more transparent, liberating and empowering people. We shouldn’t be afraid of blocking systems that don’t meet these standards, but we must rapidly embrace those that do. They can make government more strategic in how it approaches complex decisions about the highest-stakes issues, with more accurate, more granular, more up-to-date information and insights.
And this is only the beginning of what AI will be able to achieve. The pace of development and the new capabilities announced each month make it clear that the current generation of AI systems only give us a glimpse of their full potential. This is the least able AI will ever be.
To access this opportunity, government will need a coordinated strategy to put in place the necessary infrastructure, sovereign capability and skills. It will need to invest in making the right data across departments interoperable, while maintaining privacy. It will need to train its own models where necessary, such as for national-security purposes, fine-tune custom tools and build or procure applications on top of existing models. It will need to secure the computing power necessary for AI to run at scale, for everyday use as well as research purposes. And it will need to change how it hires and trains AI specialists.
None of this will be possible without working in partnership with the private sector. The computing requirements of AI mean that close coordination and cooperation with leading providers are required. The UK is also itself home to many leading AI companies. With the talent that we have, it should be home to many more in the future. The government will play a crucial role in fostering this industry if it makes the right choices and clearly demonstrates what AI can help us achieve.
For those of us in both the public and private sectors, the choices that we face today are critical for our futures. Businesses which fail to adapt to this new world will be quickly replaced by competitors. For countries, the failure is bigger – harming people’s prosperity as well as their nation’s place in the world.
The prospect might seem daunting, but for the most part investing in AI is low-risk, high-reward. Its benefits, as this paper shows, far exceed the costs – and the price of inaction may be higher still.
Tony Blair, Executive Chairman
Marc Warner, CEO, Faculty
Chapter 2
With costs mounting, backlogs growing and outcomes worsening, it should be clear to every political leader that the way government runs no longer works.
Outside the public sector, a great change is underway. The combination of massive volumes of data, ubiquitous cloud and powerful processors has created a self-reinforcing feedback loop of innovation and growth.
The latest iterations of artificial-intelligence systems – generative AI such as large language models (LLMs) – are matching humans for quality and beating them on speed and cost. Knowledge workers using the GPT-4 model from OpenAI completed 12 per cent more tasks, 25 per cent quicker, with a bigger boost in productivity for less-skilled workers. Businesses using AI tools are 40 per cent more efficient and have 70 per cent higher customer and employee satisfaction than businesses that do not.
And unlike previous generations of AI systems, which had to be custom-built, generative AI is general-purpose, opening up a wide range of applications.
In the private sector, the transformation is accelerating. Leading tech companies are reportedly planning investments of more than $250 billion in chips, compute and data centres. Corporate investment in AI since 2020 is close to $1 trillion. Adoption is soaring: within nine months of launch, ChatGPT was in use in 80 per cent of Fortune 500 companies. Spending on generative AI systems by European businesses is expected to grow by 115 per cent in 2024. By 2025, Goldman Sachs expects AI investment to reach $200 billion a year globally. By 2028, analysts expect the global market for AI to exceed $1 trillion in size.
In the public sector, profound changes are now possible. Harnessing AI tools could repair the relationship between government and citizens, put public services on a new footing and unlock greater prosperity.
This prospect should be exciting in its own right, but in reality it is the only path forward. The public sector is on its knees, with large backlogs and lengthy waits for services, a demoralised, unproductive workforce and a lack of long-term thinking as policymakers go from crisis to crisis. Adopting AI in the public sector is a question of strategic prioritisation that supersedes everything else. The UK cannot be consumed by old debates when the real issue is AI.
AI could make countless tasks performed by public-sector workers every day better, faster and cheaper. It could help them to match service supply to demand, accelerate processing of planning applications or benefits claims, upgrade investigations and analysis, communicate with citizens better, collect and process information for transactional services, model and intervene in complex systems, expedite research and support tasks, manage diaries, draft notes and much, much more.
In fact, the UK government believes that up to a third of public-sector tasks could be improved with AI. Now, TBI analysis shows that, after accounting for upfront and ongoing costs, the UK stands to gain £40 billion per year in public-sector productivity improvements by embracing AI, amounting to £200 billion over a five-year forecast.
The public sector cannot afford to leave this on the table.
To take advantage of this opportunity, this report, written in collaboration with Faculty, a UK-based applied AI company, recommends the following actions:
1. Set up a new, expert AI operation in Number 10 to join up existing teams – an AI Mission Control, headed by a dynamic AI Mission CEO with a strong mandate to drive change. Reporting to the prime minister and working closely with departmental teams, it should act as a beacon for the best and the brightest people to build a new operating model of government.
2. To give this mission real momentum and remove barriers to rapid progress, the next government should in its first 100 days:
Put the chief secretary to the Treasury in charge of digital transformation, data and AI across government (as in Australia), to work hand in hand with the new AI Mission Control and its CEO, and ensure the Treasury specifically directs departments to include proposals for AI systems in funding bids.
Ask the National Audit Office to urgently review its value-for-money evaluation approach in a way that would increase a department’s tolerance for risk of failure.
Launch an urgent review of civil-service career frameworks for the age of AI, accompanied by a surge of AI talent into departments, with streamlined recruitment, two-way secondment schemes and a dedicated graduate-entry route for AI experts.
Nominate a small number of “AI exemplar” departments such as the Department for Science, Innovation and Technology and the Department for Energy Security and Net Zero, providing funding and a clear mandate to bring their operating models and work environments in line with the best UK firms in their domains.
Enforce and fund a “Bezos mandate” requiring all government departments to provide clearly documented, secure ways to access data and functionality.
3. The AI Mission Control should build the technological foundations of a truly AI-enabled state. AI adoption in government will not succeed without the right infrastructure and the right partners to deliver it. To achieve this, the AI Mission Control will need to:
Make data interoperable: secure upfront funding to rapidly link data across government that will make the implementation of AI at scale possible, maintaining privacy and anonymity. Prioritise interoperability over the replacement of legacy systems, which can be more gradual.
Build sovereign AI capability in collaboration with trusted private-sector partners: government will need to create its own models, fine-tune existing ones or build tools on top of off-the-shelf LLMs, deciding on the appropriate approach and the best foundation model for each use case, to ensure that it can: first, train its own custom LLM for national-security purposes (which for the purposes of this report we name CrownIntel) on open and official data, and fine-tune it on sensitive and confidential information in a secure environment; second, create a ChatGB “legal advisor” tool for government by fine-tuning an off-the-shelf GPT-4 class model on legislation and parliamentary records; third, work with individual departments’ digital teams to build or procure AI tools using commercial foundation models. These tools for ministers, officials, caseworkers and analysts would be bespoke to each department’s individual use cases and could number in the hundreds or thousands.
Secure necessary computing power: first, purchase dedicated graphics processing units (GPUs) for a secure computing environment such as that of the CrownIntel model and invest in extra AI computing capabilities within the Crown Hosting Data Centres private-public partnership; second, continue to pursue favourable rates for public-sector bodies with hyperscalers such as Oracle, Microsoft, Google and Amazon, leaving the choice of final cloud provider for most applications to individual departments, encouraging greater competition in the space; third, collaborate with the national grid and hyperscalers to continuously monitor AI computing demand and ensure capacity can be ramped up where necessary; fourth, ensure that the UK AI Research Resource ramps up to 30,000 GPUs in the shortest possible timeframe.
Win the talent competition: benchmark salaries for AI-related roles to at least 75 per cent of the private-sector market rate and streamline hiring procedures, with a charm offensive to attract the best and brightest into AI Mission Control and departmental digital teams.
4. Use existing AI tools to end the backlogs and waiting lists plaguing public services, freeing them up for deeper reforms. These are tools that are already being used, but only in a handful of hospitals, schools and government offices across the UK. If implemented intelligently across the system, they could:
Bring bed occupancy in the National Health Service back to the safe level of 85 per cent not seen since the early 2010s, saving lives and freeing up capacity to deal with backlogs.
Save teachers in schools from evening and weekend overtime by significantly cutting time spent marking and planning lessons.
Clear the Department for Work and Pensions’ growing backlog for new Personal Independence Payment claims in ten months or less with better triage and prioritisation, at a total extra processing cost of less than £100,000.
Cut consultation costs across departments by 80 per cent, saving £65 million a year, and speed up decision-making in the planning system.
With these foundations in place, the next government could reimagine how the state engages with citizens, operates and makes decisions, creating a new approach to governing in the age of AI. A new model of government that is higher quality, faster and less costly.
In this report, we describe an AI-enabled model of government in which every citizen has their own digital public assistant to help manage their relationship with the government, freeing up their time.
Every public servant works alongside a team of AI co-workers and helpers, freeing them up to work on tasks that need their skills and dedication.
Every minister or policymaker makes agile, aligned, strategic decisions with the help of a National Policy Twin, freeing them up to focus on unlocking prosperity and growth.
Amid worry about the state of the public finances, a stagnant economy and crumbling public services, this moment might seem like the most limiting in living memory to be in government. In fact, considering the opportunities now presented by technology, it might yet be the most transformative.
AI makes it possible to reimagine the state. The UK can again show leadership by demonstrating to the world what it means to govern in the age of AI.
Chapter 3
For the private sector, the artificial-intelligence revolution promises greater productivity, lower costs and higher customer satisfaction. For governments, it presents an opportunity to reshape the social contract with citizens and change the trajectory of public-service delivery. The private sector is acting on this promise already – and governments must seize the opportunity at hand.
The Industrial Revolution spurred the British and other states to reimagine how they operated and reinvent their relationship to citizens. A new operating model of government – in the case of the UK, the civil service as a “Whitehall machine” – made the welfare state possible.
This model was built on contemporary innovations. Steam-powered printing presses, railways, telegraphs, typewriters sped the flow of information to, through and from Whitehall. Beginning in the early 20th century, computers exponentially increased the state’s ability to understand its citizens, the economy and the wider operational environment, and to act on those insights.
Harnessing these kinds of tools, successive governments delivered comprehensive education and health-care systems, infrastructure and housing to address ignorance, disease, want and squalor.
But as state capacity expanded, so did the scale and complexity of public-sector tasks. As an increasingly sophisticated private sector began to shape citizens’ expectations around the speed and quality of delivery, [_] the labour-intensive services provided by the public sector experienced continually rising costs amid stagnant productivity, as explained by Baumol’s cost disease.
This combination of factors put the public realm on a cyclical path downwards. As maintaining service levels gets more expensive, capital spending is delayed and salaries are held down. The need for constant firefighting in the public sector, coupled with better conditions in the private sector, makes it hard to compete for the best talent, as evidenced by record numbers of leavers and a collapse in graduate-scheme recruitment. With fewer well-qualified people in a labour-intensive sector, backlogs grow, service quality falls and pressures increase. This is the “doom loop”.
Traditional debate remains focused on two approaches to these challenges: spending more on the current model or cutting back on service provision. Yet as citizens demand better public services and the fiscal outlook worsens (not least due to a lack of long-term investment), the current model may be reaching the end of the road.
However, a new set of tools – AI foremost among them – now offer a plausible path forward: a new model of government that breaks the doom loop.
Chapter 4
AI is already deeply embedded in the fabric of our everyday lives. It recommends shows to watch and articles to read, powers voice assistants on our phones, provides directions that avoid traffic jams and flags up cancelled trains. Much of it is so seamless that we barely notice.
The same tools are reshaping how companies engage with customers, manage operations and make decisions. Salesforce’s Einstein, a customer-service tool, has driven down call times by double digits. Klarna’s AI assistant handles two-thirds of customer queries, faster and with fewer repeat enquiries. The Economist reports Nasdaq analysts are using AI to identify suspicious transactions ten to 20 times faster, while AI systems at Bank of New York Mellon are generating first drafts of briefing notes from live data overnight. In a recent IBM survey, 43 per cent of CEOs said they used AI for strategic decisions.
These are not isolated use cases. Data show the strength and pace of the response to the latest developments.
Generative AI is expected to increase productivity for private-sector companies by between $2.6 and $4.4 trillion annually.
In retail, the value of generative AI could reach 44 per cent of operating profits, or $660 billion a year. Banking would see productivity grow by 5 per cent of annual revenue – the equivalent of $340 billion in value. One analysis suggests this could boost revenue by $3 million to $4 million per employee per year. Knowledge workers using the GPT-4 model from OpenAI completed 12 per cent more tasks, 25 per cent quicker, with a bigger boost for less-skilled workers. Businesses using AI tools are 40 per cent more efficient and have 70 per cent higher customer and employee satisfaction than businesses that do not.
The private sector is alive to the opportunity and moving fast.
One sign of this is the rapid growth of investment in the essential infrastructure of AI – computing power. Leading tech companies are expected to invest more than $250 billion in chips, compute and data centres, with industry reports and public statements from Microsoft and Google that each will likely spend at least $100 billion in the next few years. In 2023 alone, the top five compute providers committed to $15.3 billion in new orders for NVIDIA’s high-performing H100 graphics processing unit (GPU) chips.[_]
Private companies are investing heavily in compute resources
Source: TBI
Private investment in generative AI companies reached $25 billion in 2023 – nine times more than in 2022. Total corporate investment in AI since 2020 is close to $1 trillion.[_]
The private sector has been fast to adopt these tools, too. Amazon’s cloud-services revenue has seen 17 per cent year-on-year growth driven by demand for AI compute. In 2025, Goldman Sachs expects companies around the world to invest $200 billion in implementing generative AI – half of that in the US. By 2028, analysts expect the global market for AI to exceed $1 trillion in size.
Enterprises are partnering with large language model (LLM) developers and building their own models, too. Moderna has deployed more than 750 generative AI tools, based on OpenAI’s GPT, with an 80 per cent adoption rate. Bloomberg has used its proprietary data to train its own research and analysis tool, at an estimated cost of $2.7 million. Morgan Stanley was an early partner for OpenAI when GPT-4 launched. PWC is investing $1 billion into AI use cases over the next three years.
In North America, generative AI spending by private-sector companies in 2023 was conservatively estimated at $3.3 billion. In 2024, it is expected to grow by 67 per cent to $5.6 billion. In Europe, spending in 2024 is expected to grow by 115 per cent to reach $2.8 billion, with France, Germany and the UK the largest markets.
And in the UK specifically, the same survey suggests at least 39 per cent of companies have implemented or are implementing generative AI solutions or established use cases – a significant change compared to 2022, when just 15 per cent of UK firms made any use of AI.
These efforts are already paying off. Less than a year since the launch of GPT-4, close to a third of companies with revenue of more than $10 billion were already creating business value with generative AI tools. JPMorgan Chase developed its own generative AI tool called IndexGPT and upgraded its expectations for business value generated by AI from $1 billion to $1.5 billion a year. Fifty-nine per cent of respondents to a McKinsey survey reported revenue increases from AI adoption in 2022.
But while the private sector is investing billions, governments are falling behind. Of notable machine-learning models released in 2023, 82 per cent were developed by industry or industry-academia collaborations and just two by governments. Although some countries are beginning to invest in chip capacity, their efforts pale in comparison to the private sector, with purchases numbering in the hundreds, not hundreds of thousands.
Number of notable machine-learning models by sector, 2003–2023
Source: The AI Index 2024 Report by Stanford University
Yet many of the tasks in the public sector mirror those AI is transforming for businesses: citizen engagement, operational management, policymaking and strategic decision-making. But governments have not yet embraced AI in the same way. They risk being left behind – or they can choose to break the doom loop.
Political leaders must seize this moment. If progress is left to tentative pilots and ad hoc experiments, the full potential of AI to reimagine the state will never be realised. They must embrace a bold vision and strategy that puts AI at the core of a new model of government.
Chapter 5
With the technology available today, we believe governments could achieve transformative outcomes within a single parliamentary term. They could eliminate some types of backlogs entirely while drastically reducing waiting times for services, turn the public sector into a great place to work and make complex, strategic, long-term planning the norm.
This is possible using AI capabilities as they exist today across a range of technologies, both in terms of generative and “traditional” narrow AI (see Figure 3). The potential impact extends from the operations of central-government departments to frontline services like health care and education or local government. If applied consistently across entire departments and policy areas, existing AI tools would have a transformational effect – whether in health, education, benefit claims, national-security research or planning applications and consultations.
How AI could transform tasks in government
Source: TBI
Eliminating Backlogs and Reducing Waiting Times to Minutes or Days, Not Months and Years
Our current predicament
Backlogs are a persistent and long-standing problem in the UK.
Simply put, backlogs emerge when there are insufficient resources (primarily, in the current model, labour) to deal with the demand for services, creating a bottleneck.
Most emblematic of this are the backlogs in the National Health Service (NHS), with millions waiting months for elective treatment. In 2023, more than a million accident and emergency (A&E) attendees had to wait for 12 hours or more for a hospital bed, contributing to more than 250 excess deaths a week. In the courts, the case backlog was more than 67,000 by the end of 2023, with more than a quarter stuck in the system for over a year.
Other backlogs are caused by low throughput of decisions on casework. Well-publicised examples of this include the delays to passport applications in 2022 and applications for asylum, where 98,500 people are waiting for decisions at a cost of approximately £4 billion a year.
Although less dependent on high-skilled labour or specialised infrastructure, these backlogs can be difficult to clear quickly. The pressure to deal with them creates a negative feedback loop: pressure to process cases drives more staff to quit, new, inexperienced workers are less productive, slowing things down even more, and political pressure can lead to attempts to game the system, eroding citizen trust and worker morale.
The time taken to process an application can stretch into weeks and months, depending on the service. New housing benefit claims take on average around 20 days to process. Applications for Personal Independence Payment (PIP) benefits, which help with some of the extra costs of long-term illness or disability, take an average of 15 weeks to be decided, with a backlog of 780,000 (including 290,000 new claims). For land-registration services, processing times can be between three and seven months for changes to existing titles and up to 20 months for complex applications such as major infrastructure projects.
The role of AI
AI systems have helped deliver remarkable improvements in addressing backlogs and accelerating casework. These are tools being used today. These gains are achieved through better prioritisation and decision-making within the existing system, freeing up capacity for long-term reform. If scaled, the impact of these tools would be remarkable.
In health care, the Hywel Dda University Health Board in Wales has used AI-powered tools, implemented in six months, to achieve a 35 per cent reduction in delayed discharges. In care coordination, Cera is accurately predicting the risk of hospitalisation, reducing hospital admissions by 52 per cent among those whose carers use it.
Scaled across the health-care system, these two innovations alone would be enough to bring bed occupancy in NHS hospitals to 85 per cent – that is, a safe level not seen since the early 2010s. This would allow the elimination of dangerous waits of more than 12 hours or “trolley waits” of more than four hours. [_]
AI tools are drastically improving productivity in other areas. By automating triage and guiding investigators to the most relevant evidence in each case, AI systems have helped National Crime Agency analysts process new serious and organised crime cases, reducing the time for processing batches of thousands of referrals from months to days – approximately a 90 per cent increase in productivity.[_]
The same level of productivity improvement, if applied to existing administrative backlogs, would be transformational. A 90 per cent increase in case throughput linked to better triage would enable the clearance of the existing backlog of new PIP claims in three months without the need to hire new caseworkers. Even a much smaller increase of 30 per cent would clear the backlog in ten months at a total additional processing cost of less than £100,000 – not by automating decisions but simply by improving prioritisation and resource allocation.[_]
Turning the Public Sector Into a Rewarding Career of Choice for Ambitious People Working at the Cutting Edge
Our current predicament
Frontline public-sector workers spend large amounts of time doing administrative tasks.
Some tasks make full use of their knowledge, judgement and communication skills. But they also spend hours on less rewarding tasks: repetitive marking or lesson planning for teachers; engaging with customer-relationship-management systems, turning meeting notes into summaries or reviewing pages of information to find relevant insights for those in delivery roles; digging through thousands of consultation submissions, drafting freedom-of-information (FOI) responses or compiling stakeholder biographies for ministers for those in policy roles.
Due to financial pressures, public-sector and civil-service jobs are often less well paid than equivalent private-sector roles. The result, as review after review has highlighted, is that recruiting, training and retaining high-quality people is difficult.
This challenge is especially pressing in areas where specialist expertise is needed. Teaching is an example of this in public services, with recruitment shortfalls in many subject areas, major retention challenges and heavy workloads. The average teacher works 12 hours a week of unpaid overtime.
The civil service itself is struggling to recruit externally, especially into specialist roles, with 37 per cent of digital, data and technology recruitment campaigns failing. The challenge is particularly pronounced in areas such as digital, data and technology: compensation for AI skills can be three to ten times less than in the tech sector. Fast Stream recruitment has plummeted, suggesting fewer graduates see it as a viable career route. In lower grades, job dissatisfaction is a massive barrier to retention, with staff most likely to leave “for better pay and benefits package” and “for more interesting work”.
The role of AI
AI systems are helping to remove time-consuming, repetitive tasks from work and ensure that people in public-sector roles can spend time on tasks where their skills make the most difference.
At the moment, full-time teachers in England work, on average, 52 hours per week. They spend around seven to eight hours on lesson planning and six to seven hours on marking.
However, experiments with generative AI in marking and lesson planning are demonstrating major time gains. By reducing these tasks to minutes rather than hours, AI tools can remove the need for teachers to work during weekends and evenings by saving 25 per cent of their time, which equates to the average 12 hours of unpaid overtime for teachers in England.
Some government departments are beginning to see the impact of AI and similar tools on repetitive tasks. At the Department for Work and Pensions (DWP), the Intelligent Automation Garage, established in 2017, has deployed projects that have collectively processed more than 19 million transactions, saving over 2 million working hours and £54 million – for example, by automating the compilation of evidence bundles consisting of hundreds of pages. A recent Institute for Public Policy Research report estimated the potential aggregate benefit of generative AI in public-sector roles at £24 billion a year. According to the National Audit Office (NAO), the Central Digital and Data Office’s (CDDO) internal analysis suggests at least a third of routine tasks in government could be replaced by AI, which would be equivalent to approximately £58 billion a year.
Together, these kinds of changes can turn the public sector into a place where people come to work on fascinating problems, apply their unique skills and use the most advanced tools.
Delivering Policy Decisions That Are Timely, Accurate and Aligned Across Departments
Our current predicament
The constant pressure of backlogs, slow and laborious service delivery, an increasingly demoralised workforce and a consistent shortfall in investment means that many departments now lurch from crisis to crisis.
The lack of long-term planning is exacerbated by ministerial churn and structural incentives for senior civil-service officials to move departments frequently. Invaluable institutional knowledge ends up lost.
The result is an endless succession of new strategies and programmes. As the Levelling Up white paper acknowledged, between 1975 and 2015 almost 40 different regional economic programmes and organisations were introduced, an average of one every year.
In industrial strategy, new plans follow one another quickly. Each covers much of the same ground and struggles to keep up with the rapid progress of technology; each looks to the past, using legacy approaches liable to do more harm than good to the economy.
This duplication across policy and departments, as well as deterring business investment, also damages the quality of public services. Civil servants’ motivation to deliver a project may erode as a new boss could decide to change the approach in an instant. Suppliers can be left in the lurch, pausing development of half-built products while the new minister decides on priorities.
At a time when government needs to be agile and responsive, its ability to test new approaches, understanding what works and correcting what does not, is highly limited.
The role of AI
AI is helping improve coordination and decision-making, speeding up feedback loops and delivering more accurate and comprehensive analysis of options. Decision-makers can model and intervene in complex systems, run real-time evaluation of policies and understand public sentiment in detail.
Verian, a leading social research company that provides citizen insights to policymakers, uses generative AI to summarise transcripts, improving both the breadth and quality of large-scale research. The use of AI tools has led to a near seven-fold increase in efficiency, with analysts reviewing 20 transcripts a day instead of three.[_]
According to the Incubator for Artificial Intelligence (i.AI), working through 30,000 responses to a consultation, analysing the data and writing a report requires a team of 25 analysts for three months. With a similar efficiency improvement, the same process could be completed by a team of this size in 12 working days. Decisions could be made much more quickly while taking full account of public views. Across the approximately 750 consultations run by the government each year, as much as £65.8 million (80 per cent) could be saved.[_]
AI is also transforming the way new policies are designed and tested. During the Covid-19 pandemic, the NHS used machine learning to compare the impact of using 111 calls as an alternative to A&E attendance in pilot regions against generated “counterfactual” scenarios. This made it possible to observe the impact in real time and make rapid decisions about further roll out.
The same type of tools would drastically improve policymaking, helping to avoid months and sometimes years of delay and allowing for rapid course correction. According to the most recent data, only about 10 per cent of the government’s major projects have a “green” Delivery Confidence Assessment rating, with 183 rated “amber” – suggesting the benefits of more accurate planning and real-time tracking of delivery and impact would be very significant.
Chapter 6
Government departments are typically organised vertically, by area of responsibility – education, benefits, transport and so on. Some departments have large citizen-facing functions (for example, DWP), others primarily interact with businesses (for example, the Department for Business and Trade (DBT)) and some are more focused on policy (for example, the Department for Science, Innovation and Technology (DSIT)).
Despite these differences, the jobs to be performed by most departments are similar and involve the management of different flows of information that are necessary for the work of government. Broadly, they can be grouped into:
Citizen-engagement flows: providing information, distributing or accepting citizens’ payments and providing non-financial transactional services.
Operational flows: processing casework, fulfilling legal obligations such as responding to FOIs, recording, managing and sharing data, and running procurement processes.
Decision-making flows: developing new policies, monitoring and improving existing policies, and ensuring broad operational awareness with up-to-date information as well as forecasting activities.
Today’s AI systems are already capable of transforming the way these functions are carried out. As new models are trained, tested and launched, the capabilities of these systems will only grow. What is needed is a strategic vision of the impact this would have and the kinds of tools or experiences that would deliver this impact.
This vision should include a focus on addressing known shortcomings of some of today’s AI systems. Government should ensure that systems are free of bias, that citizens’ trust is earned and taken seriously, and that a human is always accountable for decisions taken with the help of AI.
Models need to be trained on appropriate data sets that are representative of the UK population, including linguistic preferences. Explainability is a must. Dedicated models should be deployed to constantly monitor decisions for unfairness or creeping bias, so any problems can be spotted and addressed quickly.
TBI has previously written about the steps the government should take to ensure AI deployments are safe and sustainable. These are important but solvable matters – and worth solving for the impact that AI can have on our lives.
Next, this report describes three AI-enabled approaches to changing the daily reality for citizens, civil servants and policymakers respectively. The UK is used to ground these examples, but the approaches are applicable in other contexts, too.
In isolation, each approach would have a powerful impact on target users. Collectively, they could transform government operations across functions and departments to create a new model of government, harnessing the full potential of new technologies to deliver better services, faster and at lower cost to the taxpayer.
Transforming Citizen Engagement With Government
2024: What AI Can Do Now
Citizens’ first entry points to government are the websites, phone lines, post boxes and buildings through which they access vital services, whether these are transactional, financial or providing information. Lowering the effort, friction and cost of this engagement – and in particular reducing inequality of access across the population – is a very achievable ambition for AI, particularly new generative AI models. Across the world, government at all levels are introducing services that take advantage of these tools to support citizens.
Streamline access to public information
A basic job of government is to explain to citizens how they can access public services. This information must be communicated succinctly and directly across multiple channels. One barrier is the sheer diversity of citizens, who have individual needs, lives and information diets; it is difficult to reach each person with the right message. Generative AI offers the opportunity to provide more personalised information services to citizens across existing and new channels, adopting the format, style, timing and even language necessary to meet citizens’ needs.
In use today: South Korea’s Seoul Talk connects citizens with AI consultants to manage enquiries and complaints related to city functions and services.
Automate transactional public services
People also engage with government through transactional interactions – tax collection or the distribution of benefits performed by multiple government agencies at both local and national levels. These services, requiring continuous updates of private information, can drain people’s attention and time. AI tools can help to reduce time costs associated with these services, both by safely identifying, linking and checking information on individuals across distributed, secure data sets and by helping citizens complete the correct information (in the right format, for example) when required.
In use today: in Portugal, the Automatic Social Energy Tariff uses safe, linked government and energy company data to identify citizens who qualify for social energy tariffs and automatically enrols them.
Combat unfairness in access to services
The digitalisation of public-facing services has enabled faster, more efficient delivery, particularly for tech-savvy citizens who are able to use smartphones and apps effectively. However, a gap has developed between people with different levels of digital confidence. Further, in both analogue and digital services, arbitrary decisions or poorly implemented models can lead to inequality and bias across demographic groups. AI tools can combat these problems. By transcribing, translating and combining information from different sources, AI can expand the number of channels available to citizens, reducing the gap between digital and other routes. By continuously monitoring and evaluating service outcomes, AI can identify existing and developing bias in services and pinpoint the root causes, helping governments to smooth access and outcomes for citizens.
In use today: voice-payment technology is being added into India’s instant payments system, which will boost financial inclusion, particularly when made available in multiple languages.
2030: A Digital Public Assistant for Every Citizen
By 2030, we envisage that every citizen could have access to a digital public assistant (DPA), a tool that intelligently suggests services, simplifies payments and provides accurate, simple and up-to-date information.
People can interact with their DPA through an app or website, by using their voice or through dedicated kiosks, always free of charge. This virtually eliminates the current dominant form of citizen-government interaction – form-filling – through a proactive “pre-approval” model for same-day delivery of services.
What the DPA can do
Look at citizens’ information to recommend services and provide “pre-approval” where they meet eligibility criteria, using recommendation engines and data matching to make forms “invisible” by default.
Use generative AI and computer vision to clarify missing information in complex cases, analysing images of document scans for relevant data and scheduling appointments.
“Advocate” for citizens in interactions with government officials, transcribing conversations in real time and responding to officials’ questions, and provide informed advice and clear explanations to demystify decision-making.
Let citizens set their own communication preferences (translating communications into a particular language or format, including integration with assistive devices), nominate a bank account connected via Open Banking for full control and opt in to be “beta testers” for government.
Allow citizens to see information held by departments, correct it and “undo” actions. Let them decide how they want data to be handled, with a full understanding of trade-offs such as the speed with which decisions are made.
What is life with a DPA like?
Source: TBI/Faculty
Today, accessing many services is a challenging ordeal. Citizens must obtain information about a service, establish whether they are eligible for benefits and grapple with lengthy forms. The wait for a response can be long and updates scarce, leaving applicants in the dark. The fear of one application impacting on another can add to the anxiety. The process can be slow, frustrating and feel like a stressful gamble.
The DPA makes these interactions frictionless and personalised. New services are targeted to eligible citizens, who receive automatic notifications and can decide how to proceed.[_] Their options, with clear explanations of decisions, next steps and other relevant services, are presented. If important information is missing, they can provide it on the spot – through text or voice, whichever works best.
Pre-approval for services means decisions feel instantaneous, but can also be easily reversed. Citizens feel informed and in control, with the DPA as their secure, transparent guide to all government interactions. Government support is tailored to their needs, responsive to changes in their lives and delivered without the waiting lists and administrative logjams people have come to dread.
DPA’s impact on departmental functions
Transactional services
The current system places the burden of proving eligibility on citizens. In some cases, this type of friction – or “ordeal” – is intentional, designed to drive down demand and generate short-term savings at the expense of greater long-term need and cost. It can be a particular barrier for people with more complicated lives and fewer years of education, who are often the ones most in need of support.
The UK has made significant progress in digitalising services, with fewer paper forms and examples of departments reusing data they already hold to minimise form-filling. For example, straightforward tax returns are effectively automated by HMRC through a combination of pay-as-you-earn schemes and pre-filled self-assessment returns. But these examples remain too few and far between.
By 2030, the DPA will extend this logic across the entire landscape of services provided by government departments. It can eliminate the burden of proof by automating eligibility checks in the background, so that citizens can see ahead of time if they are pre-approved and decide whether to access the service, saving them time and effort.[_] This also saves time for government officials, cutting decision times to seconds. AI techniques can be used to accelerate the process of matching citizens to services and to explain decisions, addressing concerns over “black-box” systems.[_]
In more complex cases, generative AI and its multimodal capabilities can ensure that additional information is captured quickly and efficiently. Form-filling is replaced by an ongoing conversation. The DPA can analyse images – for example, document scans – and extract relevant information.[_] It can ask – including through live voice chat – follow-up questions, clarify details and highlight any areas of uncertainty, and generate a “likelihood of approval” score for an application.[_]
It can track and display an application’s progress and its estimated “time to decision”. If citizens need to speak to an official to resolve issues, the DPA can facilitate this by confirming their availability and assigning the citizen’s case to an available, qualified civil servant, using AI to match capacity and demand. During the interaction, it can transcribe the conversation, providing timely and legible advice to the official based on the applicant’s circumstances – effectively advocating for them.[_]
Citizen payments
Financial interactions between the citizen and the state are increasingly digitalised, but in many cases remain opaque and slow. In the UK, the universal credit platform has streamlined some of the process of receiving benefits – for example, by showing when payments are expected to be made. HMRC can also be proud of its payment infrastructure, which proved its utility in the early days of the Covid-19 pandemic when it was repurposed in a matter of weeks to support the furlough programme.
However, there is no unified view of the outgoing and incoming transactions for a citizen across the landscape of services, with different platforms used to manage universal credit (UC), tax-free child care, tax payments, tax credits and so on. Receiving money can be a lengthy process, with a five-week wait for the first UC payment. Self-employed citizens in particular can struggle as they need to report changes in circumstances and the system is not well-adapted to the irregular nature of the income they receive. Ultimately, the government’s financial services lag far behind the many user-friendly, Open Banking-enabled fintech apps in the private sector.
By 2030, the DPA will simplify financial transactions between citizens and the government. The DPA gives citizens a single overview of their benefits and obligations – effectively, their “balance” in relation to government – to give them confidence in their financial situation. Citizens nominate a bank account to receive money quickly, set up automatic payments or opt to approve each transaction manually.
AI recommendation engines signpost users to useful tools (for example, some citizens prefer to use independent, anonymous benefits calculators) or organisations that can help them, such as Citizens Advice. The single overview also minimises the risk of inadvertent fraud – for example where citizens may not be aware of the impact of changes in circumstances or by flagging overpayments – through anomaly detection. All such flags are fully transparent to citizens, with clear explanations generated in each case and clear routes to review.
Citizens can choose whether to connect their bank accounts via secure Open Banking protocols, speeding up payments and streamlining the reporting of self-employed income or changes in circumstances as well as creating opportunities for the government to provide useful advice.[_]
Citizens can also opt in to receive personalised advice: for example, by sharing their household energy-usage data via an app integration, they could receive tips on energy-saving measures like lowering the boiler temperature or how to access government support for heat-pump installations or insulation. A self-employed worker could ask to have their likely tax bill calculated with predictive AI technology and see suggestions for interest-bearing accounts to put the money to work until the filing deadline.
Information services
Most governments rely on citizens finding the information they need by themselves. Best practice over the past decade has been to integrate the various departmental websites that emerged in the first wave of government digitalisation.
The UK’s Government Digital Service (GDS) led the way in this area, with GOV.UK rightly recognised and widely emulated. But even the best government websites are information-dense and can contain many thousands of pages. Information is not always easy to find and is often difficult for a non-specialist to read. This becomes a particular challenge when rules and regulations from different departments overlap in complex ways, making it difficult for citizens to make informed decisions.
The potential of generative AI to improve the way citizens access information from government is already well recognised. Experiments are underway with chatbots that can respond to questions about content on government websites, provide customer support and even generate custom pages on the fly based on a prompt.[_] Promisingly, users find these tools helpful and trustworthy.[_] In the private sector, they are already successfully handling millions of conversations.[_]
By 2030, with a digital public assistant, citizens will have a single point of access to all the information they need, any time they need it. They can ask the DPA questions about rules and regulations, and receive up-to-date answers in plain language that include the relevant information from across the regulatory landscape. This includes clear explanation of decisions, based not only on the type of information that is published today but also, for example, on internal guidance documents about what to do in complex cases.
With generative AI, the DPA can craft responses that are accurate, up to date and personalised to users’ circumstances, as well as adjusting the presentation of information to users’ needs and preferences. For example, it can respond in the citizen’s preferred language, such as Welsh, or seamlessly integrate with assistive technology. It can also support neurodiverse users by, for example, generating text and images to create a visual story of an upcoming interaction – such as an appointment – for people with autism.[_]
Citizens can receive alerts when changes are planned to rules that affect their circumstances and immediately see the impact of proposals as well as changes in legislation.[_] As the Institute for Government noted in relation to the Parliamentary and Health Service Ombudsman report on changes to pension age, people often pay little attention to profound policy changes that affect them; presenting this information in personalised ways through a single hub like the DPA helps ensure every citizen is well informed.
Addressing citizens’ privacy concerns with PEARS
A recent survey suggests 84 per cent of UK citizens would prefer public services to be proactive and 93 per cent are happy to share their data for this purpose. However, some citizens may have legitimate concerns over the level of control and agency they have, the potential for an “all or nothing” binary where all services are fully automated and the perceived loss of human decision-making, removing the ability to address complex cases. There are also legitimate privacy concerns around a tool that might search a range of personal data to find the right information to apply for a service.
The DPA should be designed to mitigate these privacy concerns, primarily through the implementation of a PEARS (predictability, explainability, accountability, reversibility, sensitivity) framework:
Predictability: Users should always know ahead of time what to expect, with clear, pre-emptive information about next steps, expected outcomes and implications of any actions. In the private sector, there are platforms that let users see how likely they are to be approved for financial products before they apply. A similar kind of predictability and mitigation of perceived risk is possible for public services. If they wish, users should be able to see a representation of their data before it is shared with a public service, providing confidence on more complex interactions (such as a tax return).
Explainability: Users should always be able to receive an immediate explanation of any decision and the reasons behind it. This should extend to approvals and recommendations, not just rejections. Any use of citizens’ data should be transparently recorded. AI models can already interpret and explain, in plain language, the factors that went into an automated decision, as well as check for bias. These tools should be used widely in citizen-facing contexts.
Accountability: Every decision, whether automated or not, should have a named human official who is accountable for it. Users should have clear, easy ways to speedily escalate any concerns they may have for review. Systems that are not “human-in-the-loop” should be “human-near-the-loop”. With AI systems freeing up officials’ time, this kind of personalised attention becomes possible. Conversely, an individual’s DPA is accountable to them and not to the government: it is a space of deliberative privacy to ask questions and prepare interactions with government services. Only the minimal amount of additional data is shared by the DPA to help citizens access services and only with the citizens’ consent.
Reversibility: Errors should be minimal and quickly rectified. Systems for decisions and actions to be reversed should be designed from the ground up, so that users and officials can override the system when required. Every user should have the ability to opt out of automated decisions.
Sensitivity: Challenging and complex cases should be handled with care and consideration. When eligibility is tied to sensitive personal characteristics, this must be handled tactfully. Where a user’s circumstances are atypical – for example a vulnerable person – the system should meet their needs just as well as it does for the majority of users.
Supercharging Civil Servants’ Productivity
2024: What AI Can Do Now
A central promise of AI is increased productivity; new generative AI tools in particular have already begun to multiply the output and quality of key tasks such as coding. More traditional machine-learning models can also be implemented across operational processes to streamline, evaluate and analyse complex workflows, giving more agency and capacity to managers and professionals. If marshalled effectively, these tools can have a transformative impact on public services: McKinsey estimates government-productivity improvements of 12 per cent and a wider boost of 1.2 per cent in global GDP from these emerging technologies.
Forecast demand for services
Tight public finances have deprived the public sector of capital investment for a decade. Calls to do more for less have too often done little more than pile pressure on public servants and create service backlogs. Intelligent technology offers a way out of this, by allowing operational managers to accurately forecast patterns in demand for a service and optimise their supply chains, workforce and other capital assets to increase the throughput of the service.
In use today: the Hywel Dda University Health Board has achieved a 35 per cent reduction in delayed discharges, increasing effective hospital-bed capacity, through technology that allows ward managers to optimise bed allocation and correctly forecast discharge dates.
Speed up prioritisation and triage
Government employs tens of thousands of professional decision-makers applying their judgement and experience across extensive bureaucratic processes, whether they relate to passports, visas, planning requests or transactional services. Despite the size of the public-sector workforce, the scale of the challenge is vast, with millions of applications across numerous overlapping routes, leading to historic backlogs and waiting times. Using a suite of AI tools at key junctures in these processes can increase efficiency and speed in the rote tasks that cost staff time and energy, allowing them to focus attention on the high-value task of scrutinising complex or high-impact cases. AI can be used to pre-check applications for the correct information, triage cases by calculating complexity and routing them appropriately, automate low-risk repeatable tasks, identify important information, summarise key features of cases and conduct quality assurance on cases using anomaly-detection tools.
In use today: investigators at the National Crime Agency have achieved a 90 per cent reduction in case-processing time (from months to days for batches of thousands of referrals) by automating the triage of inbound intelligence and guiding officers to the most relevant evidence in each case.[_]
Upgrade investigations and analysis
In an operational environment it is critical that the analysis driving decisions is not only high quality and comprehensive but also timely. When literally every second counts, it is possible to deploy AI on streams of real-time data to drive insights at a pace and scale that would be impossible to replicate with human analysis. This not only accelerates operational analysis but allows threats to be identified more promptly. AI can also power predictive analytics, aiding law-enforcement agencies in pre-emptive measures to prevent criminal activities.
In use today: the cross-government Counter-Disinformation Data Platform allows government analysts to quickly comb through publicly available data, identify and monitor disinformation narratives, understand the behaviours and techniques that are amplifying them, and identify attempts to artificially manipulate the information environment.
2030: A Multidisciplinary AI Support Team (MAST)
By 2030, we envisage that the civil servants who carry out the work of government could be guided and supported in their day-to-day tasks by a Multidisciplinary AI Support Team (or MAST) platform. Rather than a single system, MAST is a platform that integrates a wide range of AI-enabled tools, which can be developed by central-government teams, individual departments or external vendors, to make officials more productive.
Each official has their own configuration of MAST built on the tasks they perform. The platform connects these tools to suggest the official’s next task based on their expertise, level of responsibility and availability. Key to its operation is the principle of “earned autonomy” for AI systems. With AI as not just a co-pilot, picking up parts of tasks, but a co-worker completing its own tasks alongside staff, the civil service is seen as a workplace at the cutting edge, enabling it to attract and retain dynamic and ambitious people.
What MAST can do
Integrate a wide range of approved tools to support civil servants in day-to-day tasks, ranging from casework to data-sharing and procurement activities.
Use AI models to automate routine administrative tasks and straightforward decisions with mature, tested systems, prioritise and assign complex cases, and provide context to support better decision-making.
Provide up-to-date information on service performance, bottlenecks or emerging issues to allow officials to identify and solve problems quickly.
Enable secure and seamless information sharing across departments without accessing the raw data, retaining a comprehensive log of all actions for transparency.
Automate tasks such as responding to FOI requests by providing officials and citizens with access to open data sets and the means to interrogate them meaningfully.
Identify potential vendors for government procurement, streamline the matching of bids to desired outcomes and monitor contract performance for potential issues, disruptions or opportunities.
What is it like to work with MAST?
Source: TBI/Faculty
Today, lower-value administrative tasks occupy a significant proportion of officials’ time. Casework, a mix of digital and paper files, is largely assigned on a first-in, first-out basis. Even simple cases demand considerable time to process reading and cross-reference against guidance. Tracking and performance data is manually managed in spreadsheets, which is a monotonous and time-consuming affair. These tasks can feel relentless.
In 2030, officials will work hand-in-hand with cutting-edge technology. Freed from mundane tasks by automation, even junior staff focus on high-skill, high-impact work chosen to best fit their expertise. Apps and AI agents integrated into the MAST platform – some are off-the-shelf products, others purpose-built for the role – support them in every job.
AI helpers sort new cases, presenting straightforward cases for quick approval and setting complex ones aside for analysis. Advanced algorithms check for bias, ensuring fairness. AI doesn’t feel like a tool; it is more like having a team of digital colleagues, letting staff dive into challenging cases, speak directly to citizens and collaborate on service improvements, guided by real-time performance data.
MAST’s impact on departmental functions
Caseload management
A significant proportion of tasks currently carried out by junior staff, including processing casework and fulfilling obligations such as responding to FOI requests, are a good fit for augmentation or automation by AI systems.
By 2030, many tasks, such as most forms of data entry, will be automated to a significant degree, with image recognition used to digitise paper documents.[_] Robotic process automation (RPA) significantly cuts the amount of time it takes to complete repetitive tasks, including ones with a physical component like printing and despatching passports or bundling up physical documents where needed.[_]
The overall caseload within departments has dropped for straightforward services where pre-approval through the DPA is available. This leaves more time for tasks where human oversight is important because of significant potential impact on citizens’ lives.
To support these tasks at scale, AI systems follow the “earned autonomy” principle. When deployed to a new use case, an AI system needs to prove its accuracy before moving to each further level of autonomy, with officials working alongside.
The “earned autonomy” model for AI implementation
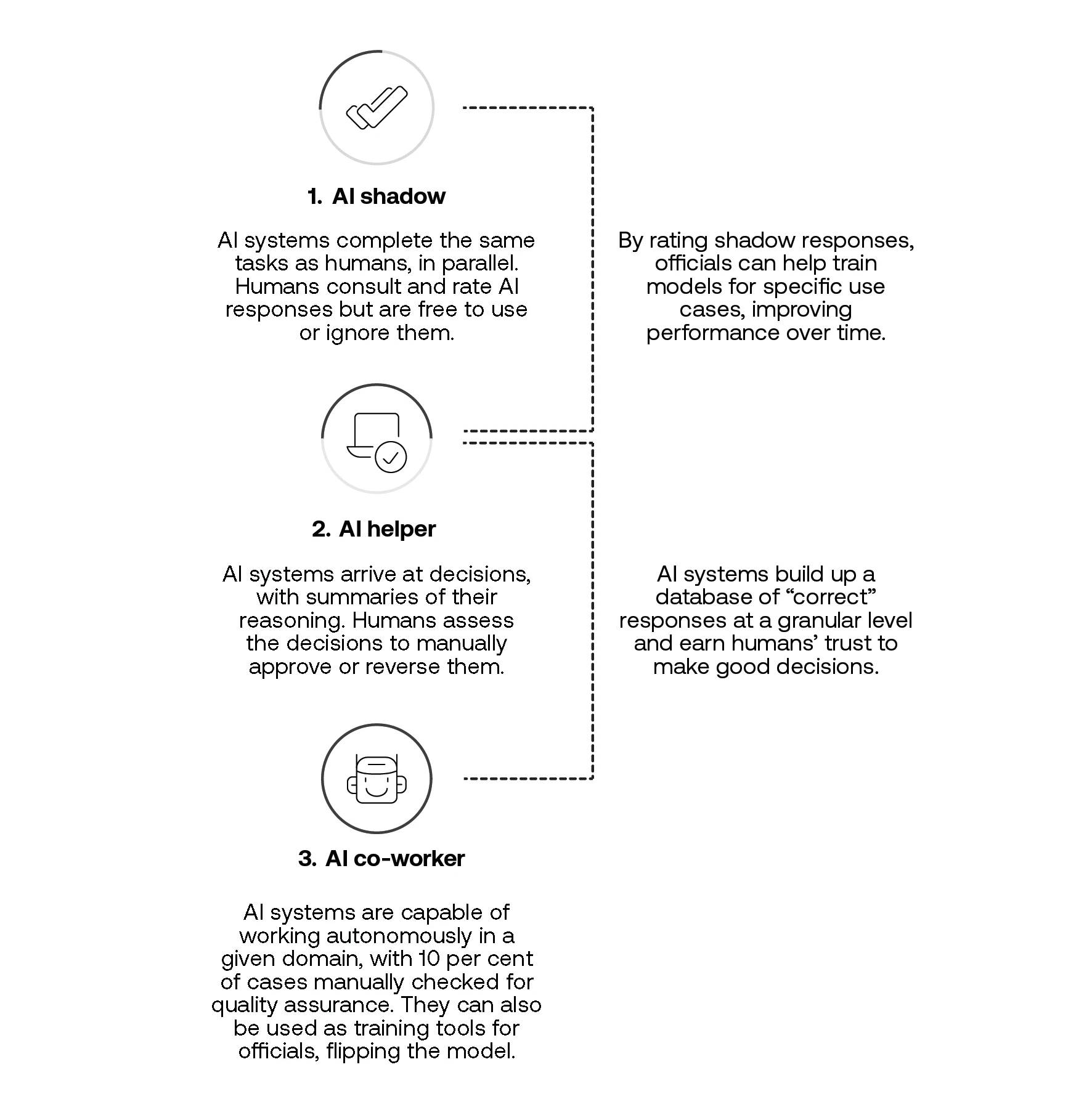
Source: TBI analysis
When a model is first introduced, it acts as an “AI shadow”. Trained on previous decisions made in a particular domain as well as synthetic data, the new tool arrives at decisions and generates clear explanations for them, with other models cross-checking the responses for accuracy and bias.
In parallel, the official responsible for the case reviews it and makes their own decision. They can consult the automated suggestion, accepting it if it matches their own or correcting it if inaccurate. This helps train the system further. In some cases, the AI system may help officials spot mistakes they themselves might make. There will be some productivity gain at this level, but it will be low.
After proving its accuracy, the tool becomes an “AI helper”. At this stage, the decision on each case in the domain is suggested by the model but signed off by an official. For each case, they read a summary of the decision with a clear explanation – also cross-checked by other models, as before – and either approve the model’s decision or flag it for further review.
This leads to significant time savings for each case, with faster decisions and automated delivery once the case is marked as resolved by the officer. A proportion of decisions are sent for manual review automatically, as are any cases where citizens have opted out of AI-enabled decision-making through their DPA (with a different standard of waiting times).
Finally, for mature systems that have proved to be accurate, greater autonomy is permitted. “AI co-workers” make most decisions automatically, with officials assigned a random sample of 10 per cent for manual review for quality-assurance purposes and as a training experience for new entrants to the civil service.
For complex cases, MAST tools triage and assign them to officials with relevant expertise and appropriate capacity. Mid-level staff spend most of their time dealing with complex cases or supporting complex decision-making. They do this with support from AI co-pilots that summarise relevant rules and regulations, provide initial judgements based on similar past cases[_] and suggest relevant colleagues to discuss issues with.
The applicant’s DPA can also act as an “advocate” in these situations, suggesting helpful information about the citizen or helping to schedule follow-up interactions. Decisions can be made more quickly and in a way that is more personalised to citizens’ needs and circumstances.
Managing data
The connected data systems necessary for these AI systems to work form the backbone of a reformed information-governance regime. Secure-by-design data sharing is the default, saving time and effort, with exceptions managed through access rights (for example, security clearance) or opt-outs for citizens. All databases are built to communicate with each other, without exception.
Every instance of data access is logged, providing accountability and assurance. To further protect privacy, the data-sharing regime defaults to what is known as “zero-knowledge proof” – that is, instead of sharing data to determine eligibility, systems generate a yes/no answer to specific questions.
The simplest example might be age or income requirements. Instead of sharing a person’s date of birth or annual income figure, the department that holds the information shares binary confirmation that the applicant is “over 65” or “earns at least the minimum required for their age and less than £100,000 per year” (as in the criteria for tax-free child care).
AI systems make this approach scalable as they can interpret individual questions – whether asked by officials or AI agents – and return a zero-knowledge proof response. This minimises the “data exhaust” – inadvertent sharing of additional information. Only the information needed to complete a task is sent across.[_] This transforms the current data-protection regime into one of data enablement.
Similar types of automation can be applied to transparency in government: the beneficial but time-consuming Freedom of Information Act regime.
Currently, responding to FOI requests requires a significant investment of time from officials to find and format information as well as make decisions about what can and cannot be shared, often inconsistently. Rather than deal with individual queries on an ad hoc basis, MAST allows departments to use open-data platforms for FOI requests, using the same mechanisms as in the previous examples.
For straightforward requests, relevant data can be accessed (in summary and raw form) by citizens directly through their DPA or third-party software. For example, developers might create apps that allow citizens to summarise data across a policy area in a dashboard or to monitor discussion of an issue they care about.[_]
AI identifies complex cases at the data-request stage, for example where sensitive information may be involved, and flags it to qualified and available staff, adding the request to their task list. These staff can automatically pull together the relevant information and then exclude non-relevant or sensitive information (with each instance logged).[_]
This approach significantly reduces the burden of dealing with the 30,000 plus requests departments receive in a typical year. It can also be used to support responses to, for example, parliamentary questions or briefings for senior colleagues or ministers.
The upside is that sharing information across departments is both secure and seamless, while the considerable burden of managing data and responding to FOI requests or similar back-office work is greatly reduced.
Procurement
Procurement is a significant area of activity for government departments, accounting for more than £300 billion a year for the UK government. Today, public-sector procurement is slow and costly for departments to deliver and for businesses to participate in, particularly for small and medium-sized enterprises (SMEs).
To reduce risks and save costs, departments prioritise bureaucracy over innovation. Large vendors with dedicated procurement departments have an advantage not because of their products or services but because they can dedicate resources to getting bids in the appropriate shape. A bias towards large upfront investment makes departments reluctant to change tack when contracts are not working, limiting scope for iteration, despite evidence that smaller, more frequent contracts are more likely to result in programme success. A focus on cost over quality encourages a race to the bottom.
By 2030, the MAST platform can integrate tools to support officials at different stages of the procurement process, from need and vendor identification to bid evaluation and contract monitoring and management.[_] With AI analysis of large data sets on economic activity and past contracts, departments can reach out directly to organisations that meet different thresholds for risk, the vendor’s financial health and track record. Bids can be focused on outcomes rather than solutions, widening the net for potential suppliers, and rely less on bidders’ ability to fill out lengthy forms (likely to be disrupted by generative AI systems in any case).
Vendors, in turn, streamline the process of putting together a bid with AI-generated responses and receive an immediate assessment of their fit prior to its submission, demystifying the procurement process for SMEs.[_] Departments can streamline and accelerate evaluation processes, freeing up time for collaboration with private-sector organisations to better define requirements, set ambitious and achievable goals as well as give deeper consideration to more diverse forms of evidence such as demonstrations or examples of past impact.[_]
Specialised areas of procurement – such as high-risk innovative products for trialling through an Advanced Procurement Agency – are supported by customised tools instead of relying on platforms built for the traditional approach.
For existing contracts, AI systems track performance, using predictive analytics to identify potential supply-chain issues, disruptions or opportunities, assisting in strategic planning and improving resource allocation. Real-time visibility over the entire supply chain enables departmental officials to track the movement of goods, monitor inventory levels and respond to disruptions promptly.[_]
This injects much-needed transparency and accountability into supply chains, giving government greater oversight of where and from whom key products are originating (a point of concern in the defence industry, for instance, with the purchase of technology and rare-earth metals).
Improving Decision-Making for Ministers and Senior Civil Servants
2024: What AI Can Do Now
Making decisions that improve the public realm, balancing competing interests and risks, is civil-service policymakers’ vocation. Despite the expansion of data, analysis and public-feedback tools in the past decade, considerable capacity is still absorbed in crafting a small number of options for decision – with more information often making this process more complex and time consuming. AI models can help to integrate, synthesise and analyse these data on behalf of policymakers and decision-makers, changing assumptions and scenarios to quickly calculate impact and monitor delivery.
Model policy systems and evaluations
The centre of government often needs to coordinate fast-moving emergencies or high-priority policy areas. But effective decision-making is hampered by a lack of up-to-date, granular information about events on the ground, and slow and indirect feedback loops between decisions and action. AI technology can resolve these shortcomings. Decision-makers at the centre can see frontline data across the country in near-real time. They can forecast what will happen next and simulate the impact of different decisions before implementing them. These tools enable the centre to take control of high-priority agendas and in times of crisis to radically improve decision-making.
In use today: during the Covid-19 pandemic, the NHS Early Warning System built by Faculty gave NHS Gold Command (the NHS’s crisis-management structure) the ability to see real-time and forecasted patient demand and resource capacity for all 215 NHS trusts, down to individual bed availability, every day, enabling resource allocations to be made across the system.
Accelerate research and support tasks
Policy officials do not spend the majority of their time researching and testing policies to surmount policy objectives. Instead, most capacity is dedicated to support tasks, such as preparing briefings for seniors and ministers; responding to FOIs and parliamentary questions (PQs); poring through consultation responses and searching for new evidence from publications and international studies. AI tools can already automate, enhance and improve all of these tasks; they can increasingly tailor outputs to the needs and style of individuals and policy areas.
In use today: the Redbox Copilot is a tool being trialled by the UK government to search through government documents, allowing users to ask questions and summarising answers into tailored briefings. The development roadmap includes integrating parliamentary records, legislation and GOV.UK content into the tool.
Understand public opinion in detail
Complex democratic decision-making requires an understanding of public sentiment – one that cuts through media noise and narrow interests to understand changing views and prioritisation across areas, groups and communities. AI can enable rapid, real-time aggregation, analysis and interpretation of public sentiment, drawing on a range of data channels and sources to build a complex picture as well as the tools to seek out and understand specific areas of detail. In this way, key decisions and policies can be shaped in response to the views of everyone, rather than particularly vocal individuals or groups.
In use today: Verian, a social-research company providing insights to public-sector organisations, uses tools based on LLMs to improve the data-synthesis stage of qualitative research studies, summarising lengthy interview transcripts in minutes.
2030: A National Policy Twin for Aligned, Agile, Strategic Decisions
In addition to operational duties, government departments are responsible for developing and implementing a wide range of policies. By 2030, we believe they could be using a National Policy Twin (NPT), a platform and data environment that brings together information from across a wide range of sources to act as a single source of truth for policy-planning processes across government.
The NPT is a complex “computational twin” that aggregates and processes structured and unstructured data from across government departments and agencies, cyber-physical infrastructure such as digital twins, official statistics and forecasts, and information about current rules and regulations. It is used at every stage of the development process for new policy, as well to monitor and iterate existing policies and provide broader operational awareness, including forecasting.
What the NPT can do
Provide a shared source of truth for policy development and monitoring based on approved data sets from across departments, a federated network of digital twins, and information about rules and regulations.
Model hundreds of scenarios and their impacts across policy areas, cutting planning time from months to days, and help stress-test proposals against desired outcomes, conflicting ideas under consideration in other departments or perverse incentives.
Generate on-demand briefings and summaries of the current state of play and service performance in any policy area, relevant rules and regulations, and any contradictions between them.
Analyse public sentiment in different policy areas, process feedback from service users and summarise consultation responses as well as the latest research evidence on the efficacy of different interventions.
Maintain a readily available shared database of international best practice to enable rapid benchmarking of policies and proposals.
What is it like to work with the NPT?
Source: TBI/Faculty
Today, policymaking is often siloed and slow. Generating new policy options or recalculating based on new assumptions take weeks. Vital cross-departmental impacts often go unnoticed and public consultations can drag on endlessly. Policy trials, though valuable, are difficult and expensive to set up and slow to yield results.
In 2030 policymakers, using the dynamic NPT system, can swiftly generate and refine policy options. This system, rooted in a common source of truth, allows real-time scenario analysis, eradicating tedious debates over assumptions. Ministers and top officials can explore and monitor policies on the move, diving deep with expert analysis as needed.
The NPT’s Evidence Hub streamlines consultations, summarising public opinion, surfacing proposals and adding global insights from a shared bank of best practice. Policymaking becomes a living process, with decision-makers actively engaging in pilot projects, adapting policies in real-time and spending much more time in the field speaking to local colleagues and residents to obtain deeper understanding.
The NPT’s impact on departmental functions
New policy development
The development of new policies is a key function of most government departments. It broadly follows the process of defining the problem and policy question, developing and comparing options for addressing the problem, consulting on those options, choosing a policy solution, designing a delivery mechanism and finally testing, implementation and evaluation. It is worth noting not every step is always followed and the process is rarely linear.
At each stage, policymakers are hampered by the difficulties of accessing information they need to make decisions, collaborating with colleagues across government, gathering and making sense of the right range of external views, and iterating on solutions rapidly and effectively. For example, generating a range of solutions to consider takes significant time, limiting both the number of options under consideration and the ability of officials to respond to feedback with new proposals.
By 2030, policymakers considering new policies can use the NPT to rapidly pull together, with plain-language requests to the system and clear visualisations, a comprehensive picture of the current state of affairs, deepening their understanding of the problems at hand.[_] This picture can be informed by current and historical data as well as qualitative insights – for example, citizen sentiment, captured through interactions with the digital public assistant, past or ongoing consultations and open sources such as social media.[_]
This picture helps policymakers spot gaps in the evidence, rapidly commission research to close them and recommend experts or organisations to involve in the process. The NPT also highlights and summarises the current rules and regulations governing an area of policy, including those developed by other departments, and even highlights potential contradictions between them.[_]
With a policy question and intent defined, the NPT supports policy professionals in generating and rapidly modelling the impact of a wide range of potential solutions, based on a set of assumptions about underlying data shared by all departments including the Treasury.
By vastly reducing the time needed to create a scenario, it allows for the comparison of hundreds or thousands of options versus four or five today, ranking them by potential impact, cost or different trade-offs.
In working sessions with colleagues, citizens, experts or ministers, the NPT can respond to live questions, re-running calculations to illustrate the impact of different suggestions. All of this reduces the cost and increases the value of engagement with stakeholders, opening up the consultation process to a wider range of voices. This includes “traditional” responses, which can be processed and summarised with AI to identify common themes and dissenting voices, as well as more effective engagement with citizens.[_]
For different proposed solutions, decision-makers can obtain an accurate picture of relevant regulatory, infrastructural or capacity constraints, so they can address them through policy design or scope. This also means that budgeting to implement the policy and maintain it over the long term is more accurate, avoiding situations where ongoing costs are de-emphasised in planning, and negotiations with budget-holders including the Treasury are based on shared assumptions and modelling.
AI can support the process of stress-testing proposals for negative consequences, such as the introduction of perverse incentives. It can help ensure that policy is oriented towards outcomes, not solutions, and test whether proposals align to those outcomes.
Based on information about availability and expertise, the NPT recommends officials best placed to design and deliver new plans so that teams – including those who may work in other departments – can be put together quickly. Because it is a shared platform, it can also highlight ongoing policy development in other parts of government, de-conflicting early and encouraging cross-departmental collaboration.
Policy implementation, monitoring and iteration
In implementing new policies as well as monitoring existing ones, the NPT allows for rapid feedback loops and iteration. Decision-makers can ask, in plain language instead of building complex queries, for detailed information about the performance of individual programmes on any set of metrics.
A significant challenge for developing new policy approaches is the need to balance experimentation with the ethical implications of providing different experiences to citizens, especially when trialling something that may fail.
Through the DPA, citizens can choose whether they want to receive “default” services or to take part in pilots, public consultations in specific policy areas and more, with AI-powered recommendations and clear explanations of what to expect. For pilot policies, these pools of beta testers can be engaged in minutes, and their user journeys and views analysed, including with A/B testing that compares experiences of using different versions of a service to identify the best version.
The government can maintain, through the NPT, a shared database of examples of good practice from overseas, to benchmark policies against and implement domestically.
The impact of existing policies can be tracked years into the past and compared against assumptions made at the time of the policy development. Their design can be compared against evidence of best practice or the evolving consensus on “what works” among researchers. Rapid evaluation against modelled “counterfactuals” lets officials assess impact in real time and make course corrections as needed.[_]
Inefficiencies or bottlenecks can be identified and improvements designed using the same process as for the creation of new policy. Government policy shifts to a “move fast and fix things” model where problems can be resolved early and experiences improved continuously, creating far better outcomes for citizens much more quickly.
As a result, decision-makers have at their fingertips easily accessible, up-to-the-minute information on their area of responsibility. They can query it in plain language and receive high-quality, rapid briefings on issues in a matter of minutes. Chasing for updates on key performance indicators is a thing of the past. Their time is freed up to focus on longer-term strategic thinking, instead of fighting yet another crisis.
Long-term operational awareness
Finally, the NPT’s blend of data and regulatory awareness makes it easy to spot new opportunities or threats and generate new evidence. The NPT enables long-term forecasting to stimulate new ideas, explore the implications of global events, such as the launch of a new technology, and assess the impact of emerging geopolitical risks – for example, on energy security or supply chains.[_] This allows officials to develop, but more importantly keep up-to-date and rapidly implement, contingency plans – something many governments struggled to do at the start of the Covid-19 pandemic.
For different emerging issues, the NPT can recommend relevant material to read or people to speak to inside government – for example, officials with prior experience – or outside, such as researchers, civil-society actors or private-sector organisations. This allows it to act as a recommendation engine for individual officials, helping them maintain broad awareness of relevant trends and build wide networks to inform their thinking.
Alongside this, the NPT includes “living evidence reviews” – meta-analyses of academic literature that update as new findings emerge and as confidence in particular studies rises or falls – and summaries of public opinion on different relevant areas of work.[_] In every case, these are personalised to the official using the platform and can flag new findings relevant to their interests as well as suggest people to speak to.
Chapter 7
Unlocking the benefits of AI will involve both initial and ongoing costs: getting people with the right skills into government, investing in data infrastructure, developing and implementing new AI tools, including specialised LLMs, and covering the ongoing costs of compute. Our analysis suggests that over five years the total cost might reach £9.2 billion, or £1.8 billion per year, with a total return in productivity gains of £199.7 billion, or £40 billion per year – a 20-fold return on investment.
AI adoption in government will not succeed without the right infrastructure and the right partners to deliver it. This starts with implementation and ensuring that data across government can be shared securely to support better citizen engagement, more efficient operations and more accurate decision-making. As AI use ramps up, government will need to provide access to LLMs, including building sovereign capability where necessary and sufficient compute capacity.
Much of this requires building strong relationships with private-sector organisations that are leading the way on AI: LLM and cloud-computing providers, chip designers and manufacturers, AI and cyber-security experts.
An effective programme of AI implementation would also require teams located at the centre of government to set the strategy, coordinate and support activities, and specialised teams in every department who would be responsible for defining specific use cases and delivering tools to support them, internally and in partnership with other organisations. These teams need to be paid at a level that is at least comparable to the private sector. If benchmarked at 75 per cent of the current market rate, the salary bill for AI is likely to be around £110 million a year, for a total of £607 million over five years assuming 5 per cent inflation.
The exact implementation cost is understandably difficult to estimate. It would include making data systems in government interoperable, the implementation of individual AI tools and staff costs.
Specifically, as use cases are identified for the implementation of AI tools, the data sets necessary to train and run these tools need to be linked through common standards. This can be challenging where the data sets in question are held in outdated formats and will incur some upfront cost. This may fall in the range of £1.25 billion to £2.5 billion, based on known examples of similar projects in the private and public sectors.[_]
Implementation work will mostly consist of building specialised tools for individual use cases within departments, based on off-the-shelf LLMs (proprietary or open-source) and integrated into the MAST platform. Some could be developed in-house, but most would benefit from external expertise and collaboration with private-sector AI firms. The individual cost of most such tools would be very low (single-digit millions, and often less) but across all use cases, thousands of these apps would need to be built.
In a typical AI implementation project, the data-infrastructure work would constitute between 50 and 75 per cent of the total cost, suggesting a total implementation cost of between £1.56 billion and £3.75 billion (including interoperability and tool design).
Some of the more specialised tasks, including those in sensitive areas such as national security, where AI can be expected to support departments, raise the issue of “sovereign AI”. Several countries have begun to invest in the development of their own models, France, India, Japan and Singapore among them. In the UK context, there are likely to be at least two areas where specialised, sovereign AI capability will be required: regulatory and legislative analysis and national-security applications.
It is important to distinguish between “sovereign AI”, in the sense of end-to-end ownership of a model and how it is used, and sovereign AI capability, meaning a level of control and confidence in the AI stack (from the training data sets to fine-tuning to the computational and serving infrastructure) that is appropriate to the use case. Not everything needs to be developed in-house, but rather governments should make strategic decisions on which components of the stack to source externally from highly trusted partners and which ones to build internally.
The government should invest in the development of two specialised cross-departmental models for legislative expertise and national-security applications. Each would warrant a different approach.
The former, a “legal advisor” ChatGB, should be based on existing commercial or open-source LLMs that are fine-tuned using open data (such as the text of primary and secondary legislation or Hansard transcripts). This would provide the model with an understanding of the regulatory landscape and let it answer officials’ questions about it. The total cost for this would be £2.5 million for a GPT-4 class model.[_]
The development of sovereign AI capability in national security would be a more complex process. In this case, the government should invest in the development of a bespoke LLM, which we call CrownIntel, trained on a combination of open-source and official data. Using this larger data set for initial training would lead to improved performance while giving officials confidence in the training process and underlying data. The resulting LLM should then be taken into a secure environment to be fine-tuned on confidential or classified data.
Rather than carry out all work in house, government should collaborate with external experts and trusted compute providers, with oversight from the national-security community. This would balance confidence in the sovereign capability with the speed of development and deployment needed. With a high degree of uncertainty, the cost of such an exercise can be assessed at up to £50 million plus £5 million per year for continuous development and fine-tuning, for a total cost of £70 million over five years.[_]
The combined implementation costs would therefore fall in the range of £2.23 billion and £4.4 billion.[_]
In addition to initial implementation costs, the additional costs of operating AI systems in government should be considered. According to modelling by the National Grid Electricity System Operator, data-centre energy use in the UK could grow from 4.8k GWh in 2023 to 17.6k GWh in 2029.[_] Recent industry analysis suggests that AI workloads within data centres are responsible for about 8 per cent of consumption today and will grow 33 per cent year-on-year.
In the UK context, this would translate to 15 per cent of total data-centre electricity consumption by 2030. TBI analysis suggests that, assuming 5 per cent inflation, over the next five years, the UK public sector would need to invest around £4.7 billion in compute capacity for AI tools, with the annual cost reaching £2.3 billion in 2030.[_]
Assuming initial implementation and ongoing costs at the top of the range, over the next five years, AI might be expected to require a total investment of about £9.2 billion, or £1.84 billion per year.
The return on this investment would be enormous. The NAO cites CDDO findings that approximately a third of tasks could be automated with the current generation of AI systems, which would be equivalent to a productivity gain of £58 billion per year.[_]
Assuming these gains take time to be fully realised and are phased in between 2025 and 2029 and adjusting for inflation, the total gains over five years would be equivalent to £199 billion.
In other words, after accounting for initial and ongoing costs over the next term of parliament, the UK public sector could stand to gain as much as £40 billion a year from embracing AI.
We cannot afford to leave this on the table.
The next government needs a plan to begin work on realising these benefits from day one.
Chapter 8
In describing the path from current to future capabilities of AI in government, we set an ambitious timeline – a vision of what is technologically feasible to build, with strategic focus and investment, by 2030.
These types of systems will not appear overnight. AI technology is evolving fast and in five years its capabilities may well look different. We also recognise that the systems we describe represent just one possible future of how AI could be used to transform the public sector. But one thing is clear: AI must be at the core of a new model of government.
Any such model will require strong foundations: secure, high-quality, shareable data sets; verifiable, privacy-preserving identity systems; modern digital infrastructure, with investment in cloud and compute capacity alongside efforts to replace legacy tech; policy frameworks to drive innovation and protect citizens’ rights; and a focus on digital skills across government and the population at large. TBI has argued consistently that these represent the digital backbone of an AI-era state and are crucial enablers for improved public services and productivity.
Individual departments will play a key role in the transition to the new model. The vision presented in this report is organised around three platforms that are not intended to be end-to-end centralised systems. Individual departments should define their own use cases and build, buy or adapt tools that integrate with them. It is essential that central teams work in close collaboration with departmental ones, going on a common, shared journey.
In fact, much of this can be built by departments today. A DPA for job seekers, “earned autonomy” casework for asylum applications and a policy twin for the electric grid are all possible. Departments should begin to think about how they emulate these examples – and many already are.
But the real power of these tools comes at scale: a DPA that connects across services, a shared productivity platform across departments, a national policy twin. This can only be achieved with a shared vision and strategy, driven from the very centre of government. Big change happens when the full authority of the head of state is behind it.
A 100-Day Plan to Kickstart AI
In the UK, the upcoming general election offers an opportunity for the new government to generate real momentum in the early days of the term. On entering office, they should, within the first 100 days:
Join up fragmented AI efforts in Number 10 and the Cabinet Office, bringing CDDO and i.AI together to establish an AI Mission Control under common senior leadership with regular reporting to the prime minister. To head this work, the government should appoint an AI Mission CEO, a dynamic leader with a strong mandate to drive change and act as a magnet for ambitious AI experts to join the government. This would address the fragmentation resulting from the rapid introduction of new AI capabilities within government across DSIT, Number 10 and the Cabinet Office, and allow coordination across strategy, policy and delivery in AI.
Make the chief secretary to the Treasury responsible for digital transformation and data across government, with a particular focus on AI. This would mirror the system in Australia (a leader in digital government), where this responsibility falls within the finance minister’s remit. The government should continue with the current practice of naming AI ministers for each department. As previously recommended by TBI, every public body should appoint a director general-level leader responsible for identifying AI use cases, as a chief AI officer or as part of the Chief Information Officer’s remit.[_] The Treasury should direct departments to ensure funding bids include proposals for AI to produce significant impacts for citizens and officials.
Ask the National Audit Office to urgently review its approach to evaluating value for money. To address the culture of risk aversion, the NAO should make it clear that, for some types of projects or programme portfolios, a certain rate of failure is acceptable and would not be considered poor value for money.
Launch a surge of AI talent into departments and commission an urgent review of civil-service careers for the age of AI. To build up internal departmental capacity, the government should set up a bespoke route into civil-service roles for people with AI skills, streamlining the application process and benchmarking pay to a minimum of 75 per cent of the market rate, establish two-way secondment schemes with leading UK AI companies and set up a graduate-entry route for AI experts through the Fast Stream and an apprenticeship scheme.
Nominate a small number of departments as “AI exemplars” that are expected to lead the way on using AI in their work and introduce a new working culture. These departments should actively engage with successful UK tech companies in their domains and replicate their best practices, down to the design of office space. Newer departments like DSIT and the Department for Energy Security and Net Zero (DESNZ) would be good candidates for this.
Introduce and enforce a “Bezos mandate” for interoperability, requiring all government digital services (internal- and external-facing) to provide clearly documented means of accessing data and functionality. The same principle should apply, with a notice period, to providers of backbone technology such as electronic patient records.
Putting AI Strategy at the Centre of Government
Governments must build the capacity to take advantage of AI as it matures – whether by following the blueprint we have presented or crafting their own. This process should be led by an “AI Mission Control” at the heart of government. This should be headed by a highly dynamic, competent “AI Mission CEO” with a strong mandate to drive change across government and who reports regularly to the head of state. The unit should include teams leading on different strands of work:
Strategy, policy and delivery coordination: a cross-functional team of policy, operational and technical experts who can develop and implement a whole-of-government AI strategy and ambitious 2030-to-2040 vision. This team should work with departments to develop concrete, well-aligned plans for AI transformation, selecting a small subset of services in each for a “learn-and-iterate” approach, with lessons disseminated across government. The team should lead the development of policy for the responsible application of AI in government, design appropriate funding mechanisms and support service redesign.
Experimentation: technologists and product experts who start putting aspects of the AI-era state in place. This team should build elements of the long-term vision that can be integrated into existing digital-government platforms and coordinate experimentation across departments, in local government and in partnership with the private sector. This iterative approach would require a cultural change in the way many governments approach IT projects today.
People: HR and learning and development specialists, skills experts and economists who define the long-term trajectory of civil-service careers. This team should consider the likely impact of AI strategy on the workforce, develop and coordinate digital skills and AI training, and consider opportunities for “earned autonomy” systems to support new entrants. The team should also monitor and enable a constant flow of relevant technical talent into departments.
Data: a team to accelerate progress towards AI-era data infrastructure that makes platforms like the National Policy Twin possible. This team should focus on moving government data sets to interoperability by design, opening up existing data sets for use by citizens and businesses, and building a single source of data truth for the government.
The exact structure and make up of such teams would vary from government to government.
In the UK, the government should set up the AI Mission Control in Number 10, led by an AI Mission CEO who reports to the prime minister and works in close collaboration with the chief secretary to the Treasury.
The AI Mission Control should quickly agree and coordinate the delivery of a small number of missions for AI-supported improvements, formulated as outcomes with clear timelines. These might include options such as “bring bed-occupancy rates in the NHS down to safe levels”, “reduce administrative backlogs by 90 per cent” and so on. A specific mission should focus on a “get fit” programme for government data so it becomes fully interoperable, with common standards.
The AI Mission Control should bring together the following teams, which are each responsible for their own areas but work closely to align activities and share lessons learned:
ALPHA (Advanced Laboratory and Policy Hub for AI) Unit to Coordinate Strategy, Policy and Delivery
This team should:
Ask every department to define, by the next spending review, and implement AI-driven changes to business-as-usual processes across citizen-engagement, operational and decision-making flows, taking the top 75 critical, frequently used services identified in the government’s 2022 to 2025 Roadmap for Digital and Data as a starting point. Work with departments to identify opportunities for large order-of-magnitude improvements to productivity and efficiency enabled by AI systems, aligned to the AI missions.
Develop guidance for the concept of “earned autonomy” for AI systems in government, including an implementation framework and success metrics for moving from shadow to helper to co-worker, and test it with the top 75 services.
Collaborate with the AI Safety Institute on a tiered procurement model for AI tools and systems, including higher-risk innovative solutions for piloting in public services and, as previously recommended by TBI, establish an Advanced Procurement Agency to oversee this. Ensure that the procurement of such systems is based on a “best affordable solution” mindset rather than a race to the bottom on costs.
Review the current approach to digital and technology spending controls to avoid holding up the implementation of AI tools, and create and maintain a register of AI systems in government.
Fund the development of smaller language models (SLMs) for improved accuracy, performance and offline availability (enabled by edge computing) for a narrow set of use cases.[_] LLMs are not economical for governments to develop themselves for most use cases and will require partnering with others to use; however, an open question remains over whether smaller models might be something that the government can build rather than buy. The ALPHA Unit should test this hypothesis.
Fund and coordinate the development of two specialised models to create sovereign AI capability: a “legal expert” fine-tuned on legislation and parliamentary records, and a “national security advisor” trained on open and official data and fine-tuned on confidential information. Both of these activities will require close collaboration with external partners to balance security and control with speed and quality.
Review the Green Book to look at mechanisms for effective and iterative funding of AI projects, with a particular focus on a portfolio-based approach that pools funding across different use cases and allows for greater tolerance of failure. This should include a new streamlined business-case process for programmes expected to deliver order-of-magnitude improvements, in line with emerging evidence that in some use cases the implementation of AI can deliver returns on investment of 200 times, as well as new mechanisms for pooling risk across a portfolio of implementations. These mechanisms should be reflected in NAO evaluations of value for money.
BETA (Broad Experimentation and Testing of AI) Unit to Create the Foundations of an Iterative AI-Era State
This team should:
As part of One Login roll-out, which links services to a single user name and password, make it possible for citizens to start setting their own preferences for use across departments for communication channels, payments (for example, a single nominated bank account) and levels of proactivity they are comfortable with. This can form the foundation of a digital public assistant in the future.
Recruit a large pool of beta testers – citizens who opt in to receive AI-enabled services and provide feedback on their quality. This should be a representative group of public-service users, drawn from recipients of the top 75 services in the first instance. These users can be identified through traditional existing service channels as well as One Login settings and the government should be transparent about the trade-offs involved, such as faster, more personalised delivery versus greater sharing of data.
For services where greater levels of proactivity might be possible – an approach that is already under consideration by GDS – implement the PEARS (predictable, explainable, accountable, reversible, sensitive) framework, using One Login as a key communication channel, with AI models to generate plain-language explanations of decisions, clear routes to escalate issues to named officials and the ability to “undo” delivery.
Work with GDS to build reusable components (such as chatbot interfaces or multimodal user experiences) that departments can readily adopt, creating the foundations of an “app store” for the MAST platform.
Run an AI innovation challenge for local authorities, fully funding a portfolio of AI programmes and providing a commitment to scale up successes. This programme should cover compute and data-storage costs as well as implementation, and include a requirement to build data sets that are interoperable by default and regular progress reviews. The portfolio approach would pool risk of failure across programmes and guarantee gradual increases in funding to those that succeed.
Launch an AI Trailblazers programme within government, running regular sessions that bring together AI practitioners from public and private-sector organisations to identify and develop use cases – a model successfully implemented in Singapore. Through this and other activities, build a horizontal community of AI champions within the Civil Service to replicate the impact of Govcamps and similar past initiatives.
GAMMA (Gateway to AI and Modern Methods in Administration) Unit to Create an Agile, Modern Civil Service Equipped to Succeed With AI
This team should:
Coordinate a review of civil-service career frameworks for the age of AI, engaging closely with the latest research on the impact of AI on skills and job families, current and potential civil servants, and private-sector experts.
Introduce a new Fast Stream scheme and apprenticeship route to train a generation of civil servants comfortable working alongside AI systems and deploy them to deliver the top 75 services. Ensure that pay for the graduate scheme remains attractive to top graduates across the country and collaborate with AI companies in the private sector to ensure training is of the highest standard.
Develop a funding model for personal IT infrastructure in government, ensuring that every official has access to modern, highly usable hardware with the latest operating systems.
Set standards for connectivity in government offices, monitor their implementation and rapidly intervene where they fall below expectations.
Work with departments to build a common culture of innovation, setting clear expectations and providing supporting training to develop relevant mindsets and operational skills. Ensure that capacity is built internally.
DELTA (Data Environments, Layers, Translation and Access) Unit to Get Government Data Into Shape and Build the National Policy Twin
This team should:
Kick off and coordinate a “get fit” mission for government data sets to follow common standards, with clear timelines and “one-time, last-time” funding from the Treasury to deliver it. Work with the Office for National Statistics on a common set of data definitions and build approved warehouses for open-source data that can be used for model training.
Support the implementation of a “Bezos mandate”, developing interoperability standards for government departments and suppliers of key data infrastructure.
Set up mechanisms for zero-knowledge sharing and integrate them with AI models to interpret incoming requests.
Sponsor the introduction of an “only-once principle” forbidding departments from asking citizens for data already held by the government and develop policy frameworks for “pre-approval” of services.
Introduce information-governance (IG) passporting, with sector bodies providing IG assurance that allows technologies to be implemented across, for example, all NHS trusts rather than receiving separate approval each time.
Move towards open-access data policies to enable the automation of FOIs in the future, by opening strategic data sets to public use, beginning with the Postcode Address File.
House a National Policy Twin Team, bringing together the National Digital Twin Programme, relevant staff from the Department for Education Policy Lab and other teams working on digital-twin initiatives across government. Continue the current effort of integrating a network of federated digital twins from across the private sector and task the team with building a general-purpose policy-development tool, starting with a computational twin of policies and regulations.
Invest in building living systematic evidence reviews for policymaking, built from the start to be machine-readable, on the basis of existing “what works” centres such as the Education Endowment Foundation.
Model the expected compute and data-storage requirements of a future National Policy Twin and allocate long-term funding to build up the necessary capacity.
Chapter 9
Highly capable AI systems are available to us here and now – and will only continue to evolve and improve. Private-sector companies recognise this opportunity and the risks of ignoring it, and are investing heavily in AI infrastructure and applications.
The government must follow suit. In every department, numerous tasks can be improved – made better, faster and less costly. Harnessing AI offers a way out of our current predicament of waiting lists, demoralised workers and embattled policymakers. At scale, AI systems in use today can free up NHS capacity, save teachers from overtime, eliminate backlogs and make government more efficient.
With the right focus and investment, the UK can become a global model for governing in the age of AI. Citizens can access services easily and efficiently. Officials can work hand-in-hand with smart AI tools, freeing them up to improve services. Policymakers can drive long-term prosperity with accurate data and real-time insights.
The prize on offer for fully embracing AI to transform the state is immense – a potential saving to the UK of up to £40 billion a year. This is an opportunity the next government cannot afford to let slip.
Chapter 10
AI is a suite of different technologies – much more than just the large language models that have captured the lion’s share of attention in the past year. Different kinds of AI can support specific tasks for individuals and organisations to complete at scale.
The following is a taxonomy of AI, broken down according to the type of task completed. These technologies are available today and are used by organisations in the private and public sectors at different scales. In assessing the potential impact of AI on the operating model of government, we focused on how these capabilities match the typical functions of a government department and the improvements they can bring.
Narrow AI
Narrow AI refers to AI systems that are designed to operate within a predefined and constrained domain, performing specific tasks with a high degree of expertise. While generative AI creates new content or data that did not previously exist, narrow AI is focused on achieving particular goals and solving specific problems using a fixed set of guidelines.
Statistical AI
Classification: assigning categories to data points.
Clustering: grouping similar data points together, for example through photo tagging.
Anomaly detection: identifying unusual data points.
Natural Language Processing (NLP)
Sentiment analysis: determining the sentiment expressed in a piece of text.
Machine translation: translating text from one language to another.
Part-of-speech tagging: identifying words as nouns, verbs, adjectives and so on.
Text summarisation: generating a concise and coherent summary of a larger text.
Language modelling: predicting the next word in a sentence.
Computer Vision
Image classification: identifying the main subject of an image.
Object detection: locating objects within an image and identifying them.
Face recognition: identifying or verifying a person’s identity using their face.
Image segmentation: dividing an image into parts to be analysed separately.
Motion detection: identifying movements within a video sequence.
Recommendation Systems
Content-based filtering: recommending items similar to those a user has liked before, based on item features.
Collaborative filtering: making recommendations based on the preferences of similar users.
Personalised recommendations: tailoring suggestions to individual user profiles.
Speech Recognition
Automatic speech recognition (ASR): converting spoken language into text.
Voice-command recognition: understanding and executing spoken commands.
Speaker identification: determining who is speaking.
Speech-to-text transcription: transcribing audio content into written text.
Voice-activity detection: detecting when someone is speaking in audio data.
Time-Series Systems
Identifying trends: detecting long-term increases or decreases in data, such as rising sales trends or declining product demand.
Understanding abnormal fluctuations: spotting cycles not tied to a fixed calendar schedule, such as economic expansions and recessions.
Spotting outliers: identifying unusual data points that deviate significantly from the norm, which could indicate errors, extraordinary events or opportunities for further investigation.
Predicting future values: estimating future data points, like stock prices or weather conditions, based on the identified patterns and relationships in the time-series data.
Simulating new scenarios: predicting the likely changes over time of key variables, based on various parameters.
Generative AI
Generative AI refers to AI applications that can generate new content, data or information that is similar to human-created content. Unlike discriminative models that classify input data into categories, generative models can produce data that is not present in the original training set. They are general purpose in nature, and outputs from the same inputs are not always exactly the same.
Text-to-Image/Video
Image synthesis: generating images that visually represent the content described in text inputs. This includes creating artworks, product designs and realistic scenes based on descriptive text.
Video synthesis: generating short videos or animations based on a text description. This involves combining elements of text-to-image generation with motion and transition models to create dynamic scenes.
Text-to-Audio
Speech synthesis (text-to-speech): converting written text into spoken words. This is widely used for creating voiceovers, reading text aloud in accessibility tools and virtual assistants.
Speech recognition: transcribing spoken language into text. While the input is audio rather than text, this technology is crucial for enabling further generative tasks, such as translating spoken words or generating text-based responses.
Music generation: composing music based on textual descriptions of mood, genre or specific musical elements.
Text-to-Text
Content creation: writing articles, stories or poetry based on prompts or outlines provided in text form.
Translation: translating text from one language to another while maintaining the original meaning and context.
Paraphrasing: rewriting text to alter its form without changing its original meaning.
Text-to-3D Models
3D-model generation: creating 3D models from textual descriptions. This can be used in game development, architecture and product design to visualise objects and environments described in text.
Text-to-Code
Code generation: producing executable code from natural language descriptions. This aids in software development by allowing developers to describe functionalities in plain English and automatically generate code snippets.
Synthetic Data
Synthetic-data production: creating artificial data that can be used in place of real data for various purposes. Examples include creating new health data to protect privacy and simulating real-world environments for driverless cars.
AI Safety
Each of these models is designed to address specific ethical and practical challenges in AI, such as understanding AI decisions, ensuring consistent performance, protecting user privacy and treating all users fairly.
Explainability
Interpreting AI decisions: using dynamic sampling to provide insights into why an AI model made a certain prediction, helping users understand the decision-making process.
Robustness
Detecting unusual patterns: employing anomaly-score technology to identify out-of-the-ordinary data points or shifts in data patterns, useful for spotting potential fraud or errors in data.
Assessing prediction trustworthiness: implementing credibility scores to evaluate how much confidence users should have in the predictions made by an AI model, based on its past performance with similar data.
Privacy
Generating safe-to-use data: creating synthetic data that mimics real-world data while preserving individual privacy, ensuring that sensitive information remains confidential when the data is used for testing or development purposes.
Sharing data privately: developing algorithms that allow for sharing data in a manner that upholds individual privacy, enabling collaborative use of data without compromising personal information.
Fairness
Correcting biases in AI: applying fairness-correction algorithms to adjust AI models and mitigate biases, ensuring that decisions are equitable without the need for extensive retraining.
Ensuring equitable AI: using model-agnostic methods to analyse and improve fairness throughout the life cycle of AI models, aiming for unbiased and fair outcomes in AI predictions and decisions.
Acknowledgments
The authors would like to thank Alex Chalmers (Air Street Capital), June Shin McCarthy, Matt Clifford (co-founder, Entrepreneur First), Mike Keoghan (ONS), Sir Patrick Vallance, Roger Taylor (former chair, CDEI) and Seb Krier (Deepmind) for reviewing the report.
Lead image: Getty